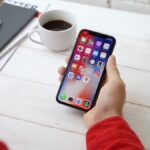
Go to the Apple App Store or Google Play Store and do a quick search for “depression” or “anxiety” and you will receive a multitude of options. Estimates of the number of mental health apps have suggested that over 20,000 such products exist (Schueller, Neary, O’Loughlin, & Adkins, 2018), with many focusing on common mental health issues like depression and anxiety. Some of these apps incorporate various evidence-based treatment elements (things like mindfulness, psychoeducation, self-monitoring, behavioural activation, or exposure) although many do not.
In fact, we see two dilemmas with widely available mental health apps:
- First, very few are evidence-based. Various reviews have suggested that about 3-5% incorporate evidence-based content, with even a smaller fraction having direct scientific evidence supporting their claims (Larsen, Huckvale, Nicholas, Torous, Birrell, Li, & Reda, 2019). In the UK, research has found no proof that 85% of mental health apps accredited by the NHS actually work (Leigh, 2015).
- Second, very few of them are used. A small minority of mental health apps account for the vast majority of all downloads (Wasil, Gillespie, Shingleton, Wilks, & Weisz, 2020), and most people who download these publicly available mental health apps never open them and rarely stick with them (Baumel, Muench, Edan, & Kane, 2019).
These two dilemmas work hand in hand. People rarely receive evidence-based content from mental health apps. Either an app does not have it, people do not download or use the app, or both.
Most of the work aimed at understanding publicly-available mental health apps has done so by defining some search terms to identify a relevant set of apps and coding these aspects of these apps on relevant dimensions such as analysing their privacy policies (O’Loughlin, Neary, Adkins, & Schueller, 2019) or coding evidence-based content within the apps (Wasil, Venturo-Conerly, Shingleton, & Weisz, 2019). This overlooks that many apps will never be used by people. As such, the authors suggest that such information should be “user-adjusted”, in that analyses of characteristics or features of apps should be considered in light of the number of people who regularly use them.
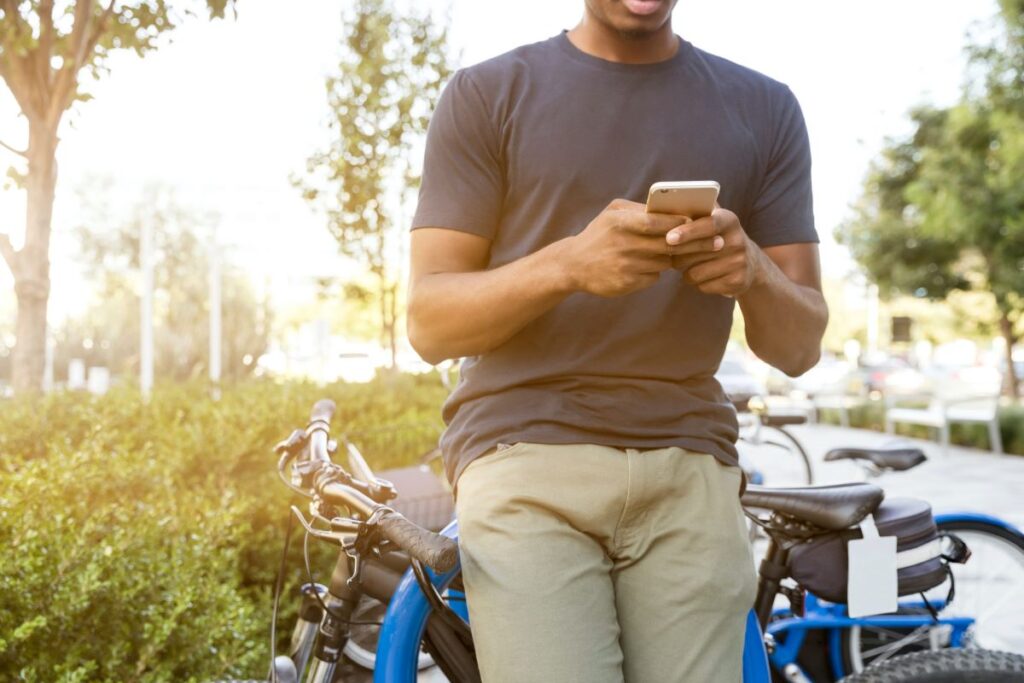
Many digital mental health apps are rarely used even when provided on app stores.
Methods
This paper built off another paper from the same group (Wasil et al., 2019). In that paper they identified 27 apps from the Apple App Store or Google Play Store related to depression or anxiety by using those words as search terms as well as additional terms recommend by the store (i.e., “depression tracker”, “anxiety relief apps”, “anxiety helper”, etc.). They then reviewed the apps to identify the presence or absence of 26 different evidence-based treatment elements.
The current paper extended the past work by performing “user-adjusted analyses.” To do so, they collected data from a mobile app analytics platform that tracks real-world app use including:
- Downloads, the number of times each app was downloaded
- Daily active users, the average number of unique users of each app on a given day
- Monthly active users, the average number of unique users of each app over the past month.
Usage data was obtained for a single month from July 1, 2018 to August 1, 2018.
Results
Two of the 27 apps reviewed (Headspace and Calm) accounted for 96% of the daily active users and 90% of the monthly active users. Headspace included five of the identified treatment elements (mindfulness, assessment, crisis management, family/significant other engagement, and stimulus control) and Calm included four (mindfulness, meditation, relaxation, and psychoeducation).
Their findings identified large discrepancies between the unadjusted and user-adjusted analyses of treatment elements. For example, although mindfulness was present in less than 40% of the apps, it was included in over 95% of the apps that had monthly active users. Other common treatment elements in apps based on user-adjusted analyses were assessment, crisis management, stimulus control, and family/significant other engagement. Whereas treatment elements more frequent in the unadjusted analyses included expressing kindness to self, self-monitoring, expressing kindness to others, behavioural activation, identifying emotions, and cognitive/coping control. This means these elements were present in apps that were available to consumers, but not those apps that people tended to use.
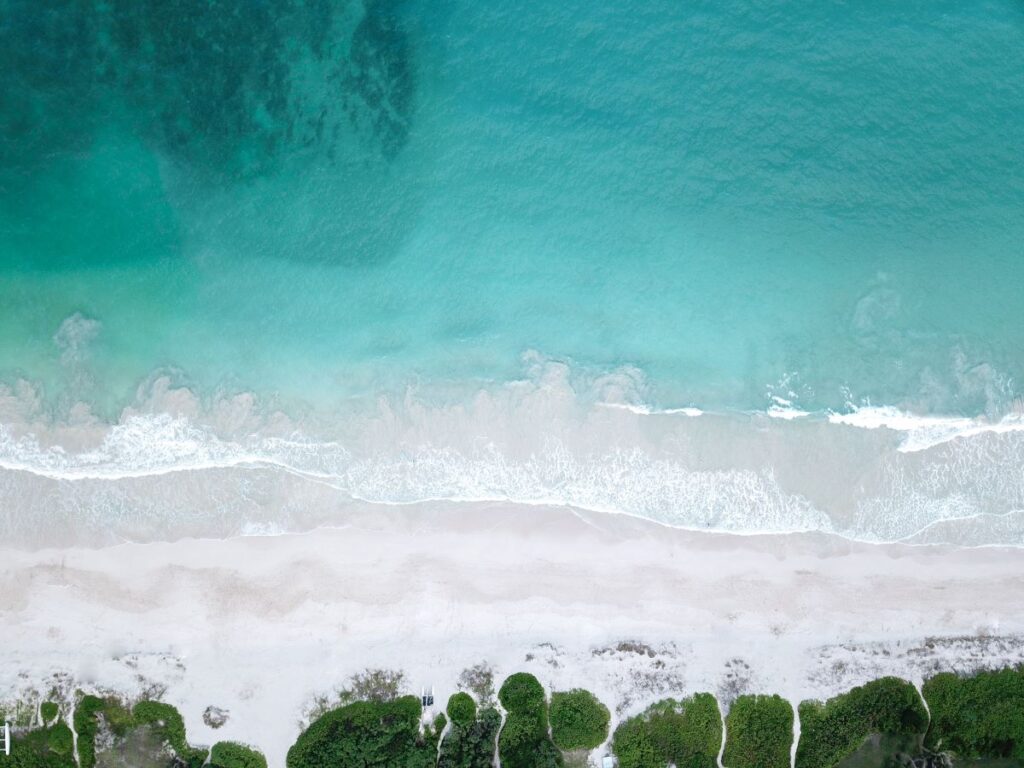
Most people flock to one of two apps: Headspace and Calm
Conclusions
User-adjusted analyses raise an important point, that understanding the impact of mental health apps in real-world settings requires not only knowing what is available, but also what people are actually using. Despite the large estimated number of mental health apps, most people (90% when considering people who used an app at least once during a month period) used only two apps. Given that both these apps contained mindfulness elements, that meant that mindfulness was present in the most used apps.
It also shows that a lot of evidence-based content translated into mental health apps is not making its way to people. Evidence-based skills like identifying emotions, goal setting, or behavioural activation were rarely identified in the user-adjusted analyses.
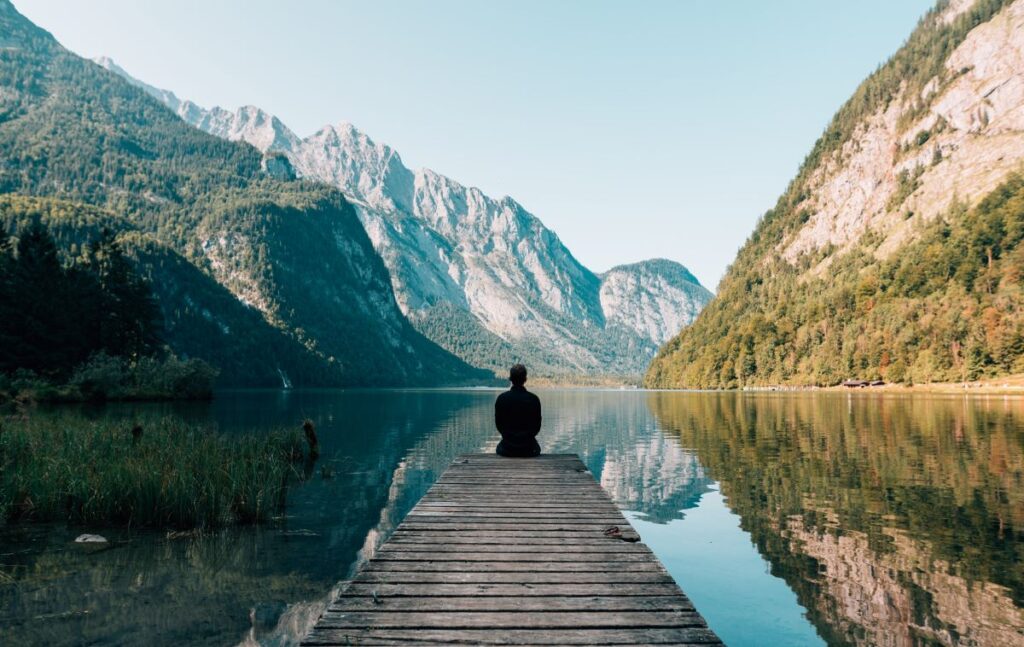
Mindfulness was the most common treatment element in the apps people used.
Strengths and limitations
Strengths
This paper reviewed a broad range of apps for depression and anxiety and explored not only what was available within those apps, but how frequently those apps were used. This data draws from real-world deployment of these apps, not clinical trials or other convenience samples. Therefore, these findings easily generalise to the real-world use of such products.
Limitations
Although both Headspace and Calm are thought of as meditation apps, the authors did not identify the presence of meditation (or relaxation or psychoeducation) within the Headspace app. Headspace, however, did have more treatment elements than Calm (5 vs. 4). As is true with many digital health products, these products change over time, and what was present in July 2018 may not be present now, however, the analyses in terms of what people were using at that time would hold true.
The user-adjusted analyses were based on one-month of app usage data. A particular product might be more or less popular in a given month expressed in both changes in downloads and use. Headspace and Calm particular, for example, have had various partnerships or marketing campaigns which might result in increases to their downloads or use within a particular month. For example, within the past couple of months Calm has been made available to American Express card owners, Kaiser Permanente members, whereas Headspace has been made available to New York state residents, Los Angeles County residents, and healthcare professionals.
Lastly, user-adjusted analyses only tell us what was present in the apps people were using, not what they actually used. Given that many apps are multi-feature, complex interventions, it is possible that people are not using all of the elements identified. Although it would be surprising if someone was actively using Headspace or Calm and were not using mindfulness elements, this might not be as surprising for other elements, such as stimulus control or crisis management. Therefore, to truly understand what people are receiving from such tools, we would benefit from user-adjusted analyses that could better explore the components people use. This has been done in other work (for example, Theilig, Knapp, Nicholas, Zarnekow, & Mohr, 2020), however this would likely require collaboration with the companies rather than an independent analytics platform.
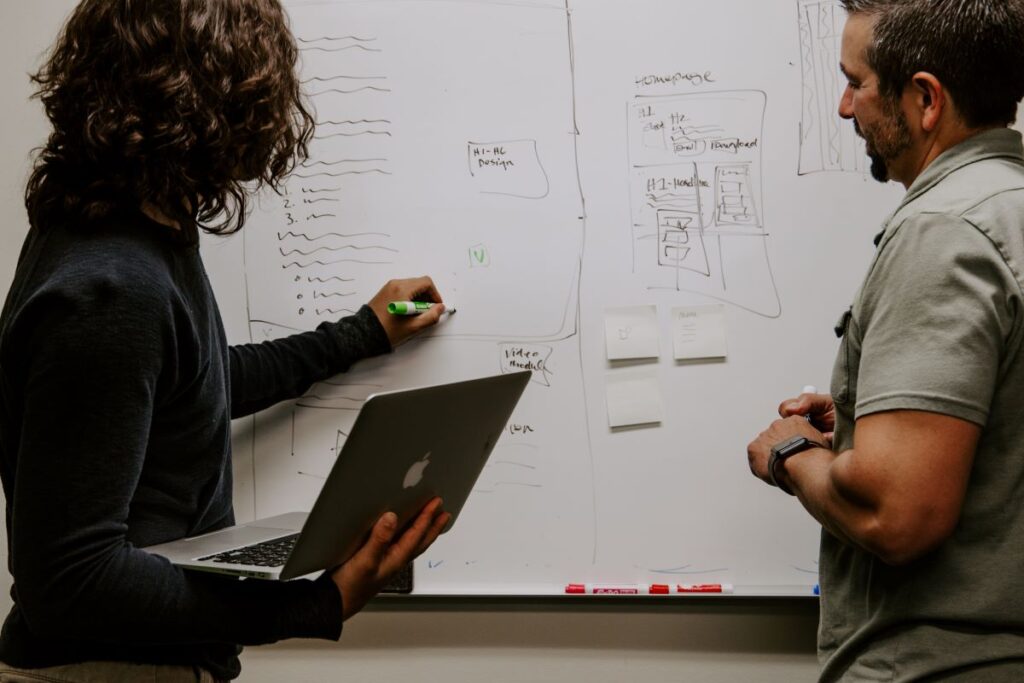
Working with industry partners might be able to provide additional insights.
Implications for practice
The findings of this study illustrate the important truth about mental health apps: if you build it, they won’t come, and as a result, a lot of potentially useful content is not being received by people. Instead, people flock to a few products. As such, we need to better understand why: Do people find these apps particularly useful or usable? Are these apps merely the best marketed and so most visible? As a provider, if such apps support the practice you are delivering you might consider ways to work in the evidence-based content into your sessions.
In the end, I’m not sure we learned a lot more about what people are using, as even within a single app various features exist, but this paper does tell us a lot about what people are not getting or what is not being successfully disseminated through current app-based offerings.
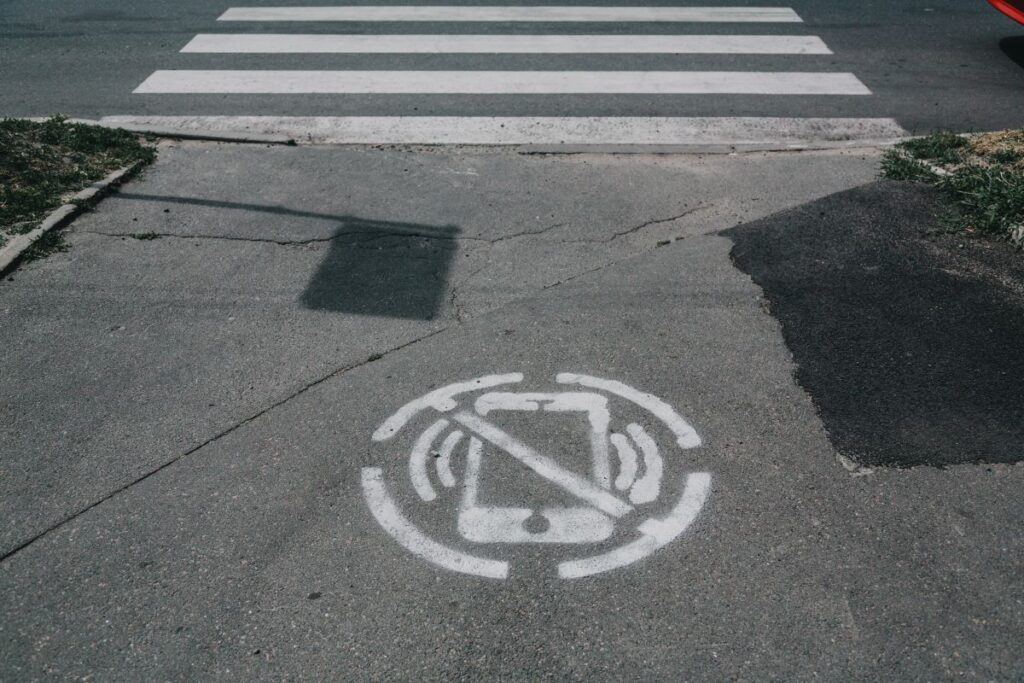
Apps that have a lot of useful treatment elements are not being used by consumers.
Statement of interests
Dr. Schueller receives funding from One Mind to direct One Mind PsyberGuide. He has also received consulting payment from Otsuka Pharmaceuticals.
Links
Primary paper
Wasil, A. R., Gillepsie, S., Patel, R., Petre, A., Venturo-Conerly, K. E., Shingleton, R. M., Weisz, J. R., & DeRubeis, R. K. (2020). Reassessing evidence-based content in popular smartphone apps for depression and anxiety: Developing and applying user-adjusted analyses. Journal of Consulting and Clinical Psychology.
Other references
Baumel, A., Muench, F., Edan, S., & Kane, J. M. (2019). Objective user engagement with mental health apps: systematic search and panel-based usage analysis. Journal of medical Internet research, 21(9), e14567.
Larsen, M. E., Huckvale, K., Nicholas, J., Torous, J., Birrell, L., Li, E., & Reda, B. (2019). Using science to sell apps: evaluation of mental health app store quality claims. NPJ digital medicine, 2(1), 1-6.
O’Loughlin, K., Neary, M., Adkins, E. C., & Schueller, S. M. (2019). Reviewing the data security and privacy policies of mobile apps for depression. Internet interventions, 15, 110-115.
Schueller, S. M., Neary, M., O’Loughlin, K., & Adkins, E. C. (2018). Discovery of and interest in health apps among those with mental health needs: survey and focus group study. Journal of medical Internet research, 20(6), e10141.
Theilig, M. M., Knapp, A. A., Nicholas, J., Zarnekow, R., & Mohr, D. C. (2020). Characteristic Latent Features for Analyzing Digital Mental Health Interaction and Improved Explainability.
Wasil, A. R., Gillespie, S., Shingleton, R., Wilks, C. R., & Weisz, J. R. (2020). Examining the reach of smartphone apps for depression and anxiety. American Journal of Psychiatry, 177(5), 464-465.
Wasil, A. R., Venturo-Conerly, K. E., Shingleton, R. M., & Weisz, J. R. (2019). A review of popular smartphone apps for depression and anxiety: Assessing the inclusion of evidence-based content. Behaviour research and therapy, 123, 103498.
Photo credits
- Photo by Yura Fresh on Unsplash
- Photo by LinkedIn Sales Navigator on Unsplash
- Photo by Michael Olsen on Unsplash
- Photo by Simon Migaj on Unsplash
- Photo by Kaleidico on Unsplash
- Photo by Марьян Блан | @marjanblan on Unsplash
To be honest, I don’t trust apps when it comes to monitoring our health condition. Sure, it can help collect data about our routine and habits, but may not be suitable to give rational conclusions and judgments. The time spent on apps should be meant for other conducive activities to boost up our mood and physical body.