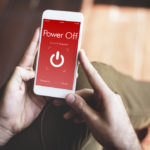
Depression; there’s an app for that. Well, there’s more like 353 on the app marketplace to be precise (Bowie-DaBreo, 2019), all with slightly different treatment approaches, visual designs and supporting evidence (or not) for their use. There’s a good reason why a growing number of researchers, clinicians and developers have dipped their toes into the digital health waters to design mental health smartphone apps.
300 million people worldwide experience depression (WHO, 2017), with the large majority never receiving treatment (Kohn et al., 2014). Smartphone apps therefore provide an opportunity to expand the reach of mental health care, making it more accessible and hopefully leading to improved outcomes. A significant number of apps targeting depression have been evaluated in randomised controlled trials (RCTs) and many have been shown to be effective in reducing symptoms (Kerst, Zielasek & Gaebel, 2019; also see Michelle Eskinazi’s and Clara Belessiotis’ 2018 blog).
Despite this, the world of health apps has a list of challenges to overcome. One of these challenges is low user engagement, and understanding why this happens. Smartphone apps will never deliver as a wide-scale treatment approach if they are not used enough or as intended. Commercial data suggest that mental health apps are opened on average once every 25 days (Baumel et al., 2019), but the picture of engagement in RCTs is less clear due to a lack of standardised reporting of mental health app engagement which hinders cross-study comparisons (Ng, 2019).
The authors of a recent review paper (Torous et al., 2019) have conducted the first meta-analysis of participant drop-out rates from RCTs of smartphone apps designed to manage depression. According to the authors, participant drop-out (not attending research assessments following a defined treatment period) “offers a standardized and practical proxy for beginning to better understand clinical engagement”. They explored a range of factors linked to participant drop-out, which in turn could be linked to user engagement with apps, and could help researchers design future trials and technology.
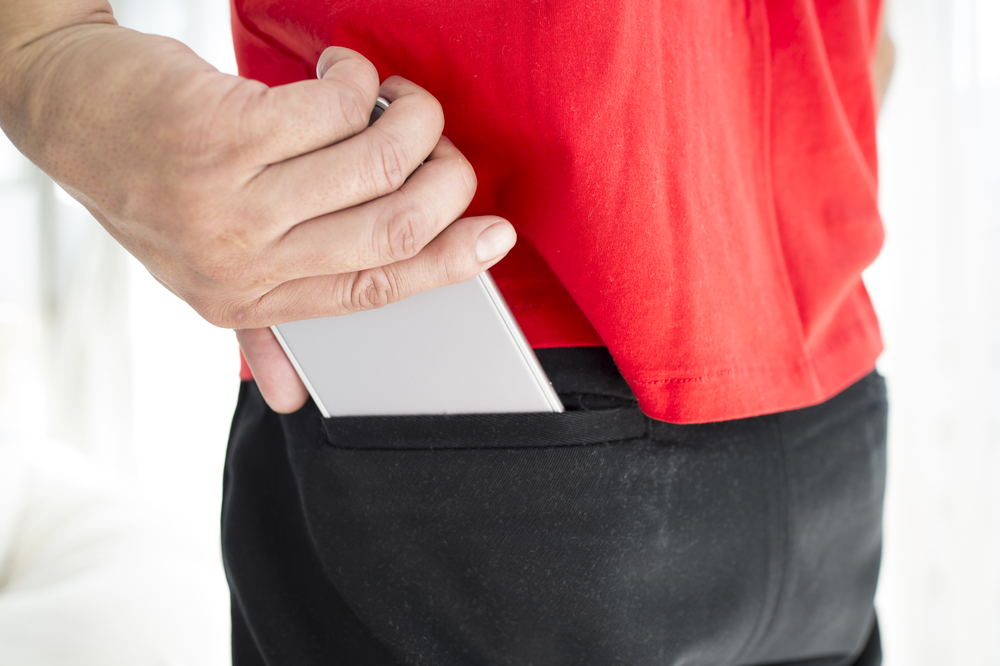
This meta-analysis investigates participant drop-out rates from RCTs of depression apps and looks to identify factors associated with it (Torous et al, 2019).
Methods
The meta-analysis was registered with PROSPERO and follows the PRISMA guidelines for reporting. The authors used search terms related to (a) depression and mental health, (b) smartphone apps and (c) RCTs to identify relevant studies in seven electronic databases.
Articles were included if they:
- Were RCTs comparing outcomes for a group receiving an app targeting depression symptoms to at least one control group
- Recruited adults over the age of 18
- Reported participant retention/drop-out at research assessments carried out after the intervention period
- Were written in English
- Were published in a peer-reviewed journal.
A clinical diagnosis or treatment of depression was not required, and all smartphone-based interventions were included regardless of treatment approach.
The authors extracted data on drop-out rates for each study that met inclusion criteria and conducted a meta-analysis to generate pooled drop-out rates (proportion of participants that completed research assessments at the end of the intervention period) for participants in both treatment and control conditions. The authors examined any publication bias, and tested for study heterogeneity. They also conducted a range of analyses to identify potential links between (a) drop-out rates of depression apps with study and app characteristics, and (b) overall study drop-out rates with study and participant characteristics.
Results
1,278 unique studies were identified in the search and were screened for eligibility at abstract and title level where 1,184 studies were excluded. 94 articles were therefore reviewed at full-text, and 18 studies met inclusion criteria. The 18 studies featured 3,336 participants in total, and tested between them 22 unique apps for depression. Within the pooled sample, 1,786 participants were allocated to use a smartphone intervention for depression and 1,550 were control participants. The intervention periods of the identified studies ranged from 10 days to 6 months.
The authors classed control groups in the included studies into three categories; (a) ‘waitlist controls’ who received no study-delivered treatment as part of the RCT, (b) ‘placebo app controls’ that had access to an alternative app that did not target depressive symptoms, and (c) ‘non-app controls’ whom received a study treatment that was not in the form of an app such as psycho-education or face-to-face support.
Participant retention for depression apps in RCTs is low, particularly in comparison to non-app controls
- The pooled drop-out rate for active depression apps was 26.2% (95% CI: 18.1% to 36.3%). Drop-out rates varied from 1.7% to 62.6% between studies and studies were highly heterogeneous (I2 = 93.4)
- After accounting for publication bias by excluding 9 studies from the analysis, the participant drop-out rate for depression apps increased to 47.8% (95% CI: 35.8% to 60%)
- Drop-out rates for participants using depression apps were not significantly different to those in placebo app conditions (25.1%, 95 CI%: 11.3% to 46.8%, p=0.91) or waitlist controls (20.4%, 95 CI%: 5.1% to 54.9%, p=0.7)
- The pooled drop-out rate for participants receiving ‘non-app control interventions’ was 14.2% (95% CI: 8.2 to 23.4) but was not quite significantly less than the drop-out rate in the active depression app group (p=0.053).
The study found no link between study characteristics and drop-out rates for depression apps
- There was no variation in depression app drop-out rates between studies that required participants to have symptoms of depression (30%, 95% CI: 20.2% to 42%) and studies where the presence of depression symptoms was not required (15.8%, 95% CI: 6.2% to 34.7%, p=0.17)
- There was no difference in depression app drop-out rates between studies that included samples of participants receiving treatment and/or have a diagnosis of depression (18.8%, 95% CI: 9.8% to 33%) in comparison to studies with non-clinical samples (31.9%, 95% CI: 20.7% to 45.6%, p=0.15)
- There was no difference in drop-out from depression apps in studies that paid participants to attend assessments (25.2%, 95% CI: 13.6% to 41.7%) in comparison to studies where they were not reimbursed (26.3%; 95% CI: 16.3% to 36%, p=0.904).
Mood monitoring and real-person feedback in depression apps are associated with lower drop-out rates, but intervention type does not impact drop-out rates
- Depression apps that featured mood-monitoring had lower rates of participant drop-out (18.4%, 95% CI: 10.9% to 29.4%) in comparison to apps where mood monitoring was not possible (37.9%, 95% CI: 23.1% to 55.3%. p=0.037)
- Participant drop-out rates were significantly lower for depression apps that featured real-person feedback (11.7%, 95% CI: 6.1% to 21.6%) than apps that did not (34%, 95% CI: 23.5% to 46.2%. p=0.003)
- There was no difference in participant drop-out between those using depression apps based on CBT (23.1%, 95% CI: 10.1% to 44.4%) and apps based on other treatment approaches (27.3%, 95% CI: 17.4% to 40.2%, p=0.7)
- Again, no difference in participant drop-out rates was found between depression apps based on mindfulness (29.3%, 95% CI: 13.9% to 51.6%) and those based on other treatment approaches (24.9%, 95% CI: 16.3% to 36%, p=0.68).
Larger studies have higher levels of participant drop-out
- A relationship was found between study sample size and drop-out rates, with larger studies having higher levels of participant drop-out (B= 0.00826, SE = 0.00415, Z = 1.993, p=0.46).
- The authors also investigated potential links between study drop-out rates and the length of study, the mean of age of the sample, and the gender distribution of the sample, but no relationships were found.
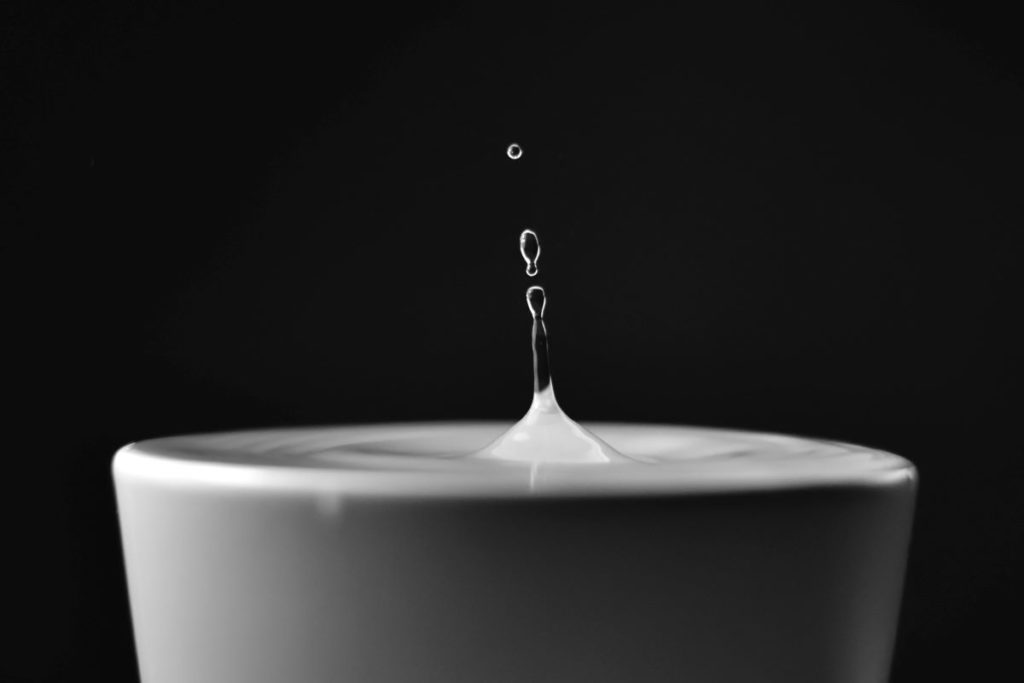
These findings suggest that research participants who use smartphone apps that target depression, have a higher chance of dropping out of research studies.
Conclusions
- Overall the results suggest that participants using apps for depression had fairly high drop-out rates, especially after controlling for publication bias
- Of interest, drop-out from depression apps had no relation to study characteristics, compensation or the treatment approach the app is based on
- Drop-out was lower in non-app controls than in participants in depression app conditions, illustrating the difficulties in user engagement with apps
- Greater retention was seen for apps or conditions where there was a human element such as person feedback.
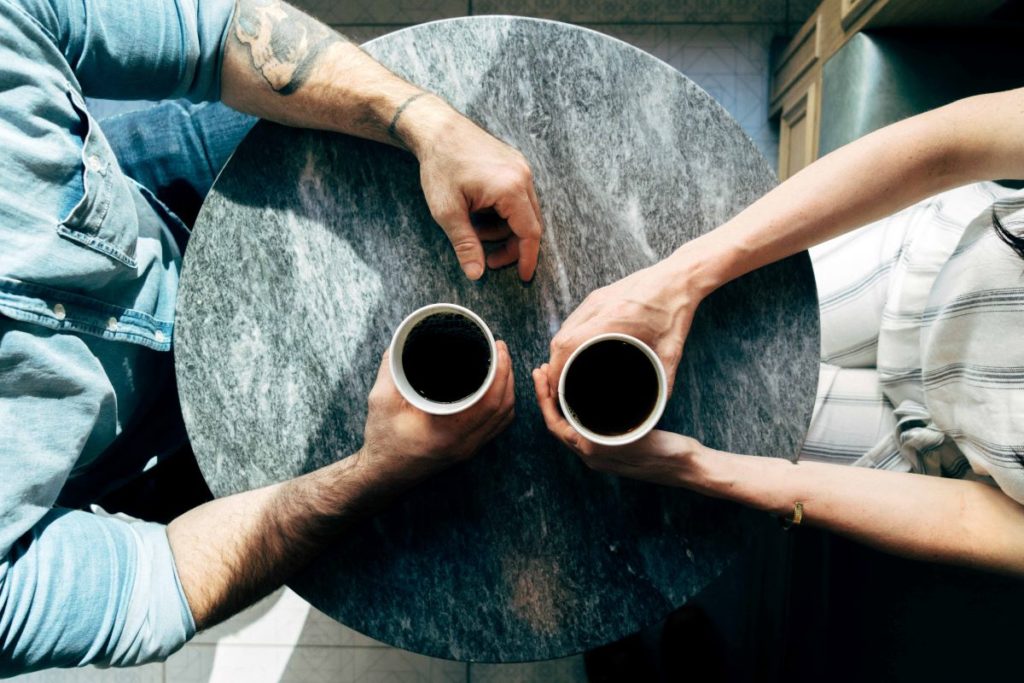
Depression apps with mood monitoring and human feedback were associated with greater retention of research participants.
Strengths and limitations
The meta-analysis by Torous et al., (2019) provides value to the literature around smartphone app RCTs, trial drop-out and user engagement. The authors conducted a comprehensive and recent search which identified important implications for designing depression apps and conducting RCTs in this area.
Only 18 studies were identified, and there was high heterogeneity and wide confidence intervals making it difficult to draw concrete conclusions regarding what characteristics of studies and apps are associated with greater study drop-out.
The measure of study drop-out is slightly problematic (a limitation that the authors note themselves). The authors state that study drop-out is a proxy measure of engagement, and therefore the findings can provide an insight on what variables might impact app use. It is unclear however what the existing relationship between study drop-out and app engagement actually is in this field, limiting the utility of the findings. Just because someone regularly uses an app doesn’t necessarily mean they are more likely to attend a research assessment, and vice versa. Regardless, this study provides a starting point in understanding drivers of low user engagement.
In addition, the type of research assessment within the included RCTs was not considered by the authors. Digital health trials employ a range of different ways of conducting research assessment such as in-person, over the phone or online and vary in length of time. The format of research assessments is likely to have an impact on who attends, and how many people typically drop-out, with perhaps a shorter online questionnaire much more preferable for participants than a gruelling two-hour face to face meeting. It is therefore feasible that differences in type of assessment between studies could partly explain variation in drop-out rates.
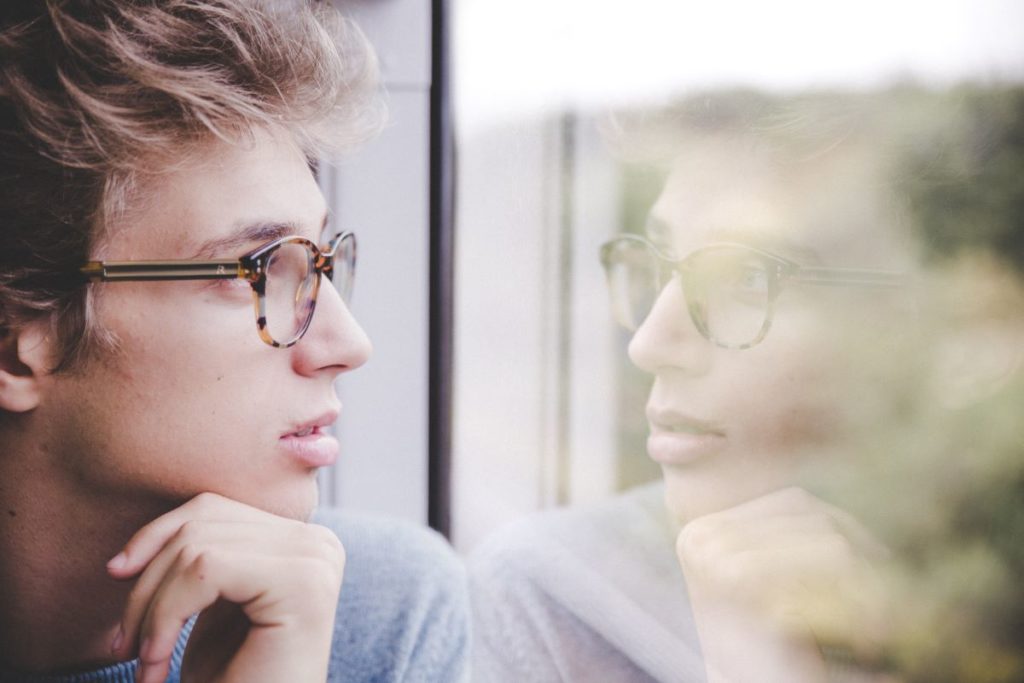
Measuring study drop-out can help researchers design better trials, but is it an accurate reflection of app engagement?
Implications for practice
For researchers the implications from this study are clear. Design better apps, conduct better trials, do more to prevent participant drop-out. High drop-out rates are a threat to the validity of the results and the progress of mental health apps. As the authors state “more research on treatment retention is essential to the growth of this field”, and a good start would be focusing on consistent reporting of user engagement with mental health apps.
High participant drop-out in depression app trials adds to the evidence that engagement with smartphones apps is tricky. To combat this, clinicians should provide guidance and support to people using apps for depression, as this could increase both app use and effectiveness (Firth et al., 2017). This will not be easy though. Providers are stretched by clinical and administrative tasks, and may lack the necessary time, skills and resources to provide effective support.
If effective support is the key to high engagement and subsequently the positive impact of smartphone apps, we need to make sure users are supported effectively and that tools are fully implemented within healthcare settings. Perhaps we need a rethink about whether the USP of apps being accessible interventions suitable for low resource settings, is ultimately realistic.
If you are thinking about using an app to help manage a mental health condition, consider discussing with a doctor or clinician. If an app on the Apple Store or Google Play makes claims that look too good to be true, they probably are. Check out the NHS apps library to find NHS approved apps that have been assessed against standards regarding clinical evidence, safety and data protection.
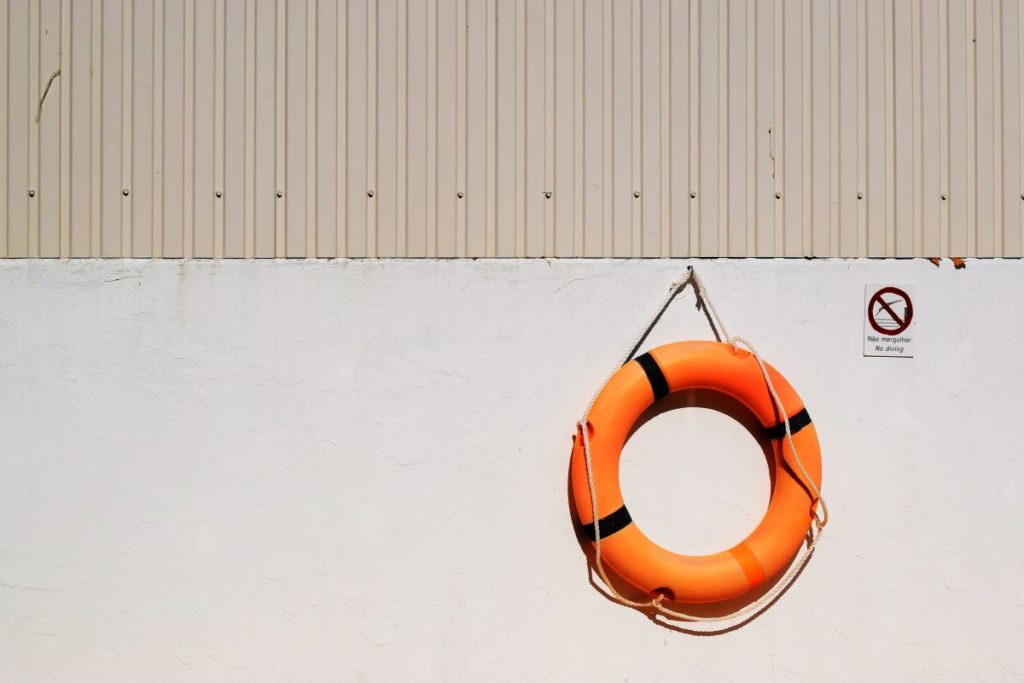
Providing support with depression apps is vital for continued study retention and most likely user engagement.
Statement of interests
Tom has worked on a feasibility trial of a supported self-management app for Early Intervention in Psychosis services.
Links
Primary paper
Torous J, Lipschitz J, Ng M, et al. (2019) Dropout rates in clinical trials of smartphone apps for depressive symptoms: a systematic review and meta-analysis. Journal of Affective Disorders. 263:413-419
Other references
Baumel A, Muench F, Edan S, et al. (2019) Objective user engagement with mental health apps: systematic search and panel-based usage analysis. Journal of medical Internet research 21(9):e14567.
Bowie-DaBreo D, Sunram-Lea S, Sas C, et al. (2019) A content analysis and ethical review of mobile applications for depression: Exploring the app marketplace.
Eskinazi M, Belessiotis C. Smartphone apps for depression: do they work? The Mental Elf, 22 Mar 2018
Firth J, Torous J, Nicholas J, et al. (2017) The efficacy of smartphone‐based mental health interventions for depressive symptoms: a meta‐analysis of randomized controlled trials. World Psychiatry. 16(3):287-98.
Kerst A, Zielasek J, Gaebel W. (2019) Smartphone applications for depression: a systematic literature review and a survey of health care professionals’ attitudes towards their use in clinical practice. European archives of psychiatry and clinical neuroscience.
Kohn R, Saxena S, Levav I, Saraceno B. (2004) The treatment gap in mental health care. Bulletin of the World health Organization. 82:858-66.
Ng MM, Firth J, Minen M, et al. (2019) User engagement in mental health apps: a review of measurement, reporting, and validity. Psychiatric Services.
World Health Organization. (2017) Depression and other common mental disorders: global health estimates. Geneva: World Health Organization
Photo credits
- Photo by Santiago Lacarta on Unsplash
- Photo by Joshua Ness on Unsplash
- Photo by Laurenz Kleinheider on Unsplash
- Photo by Matthew Waring on Unsplash