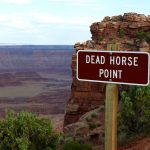
Cognitive bias modification (CBM) is a computerised intervention that trains people to overcome the automatic cognitive processing biases that play an important role in the development and maintenance of psychological disorders.
The types of biases targeted by cognitive bias modification include:
- Attentional bias (when stimuli related to the disorder capture the attention)
- Approach bias (when stimuli related to the disorder evoke approach behaviour automatically)
- Deficits in response inhibition (when stimuli related to the disorder impair the ability to control behaviour)
- Interpretive bias (when ambiguous stimuli related to the disorder are interpreted in a way that exacerbates symptoms).
CBM has been applied to various psychological disorders including emotional disorders (e.g. depression and anxiety) and substance use disorders (addictions).
There is some debate about the efficacy of CBM for emotional disorders:
- One meta-analysis (from the authors of the current target paper) concluded that CBM may have ‘no significant clinically relevant effects’ (Cristea, Kok & Cuijpers, 2015) in this domain,
- Whereas others meta-analysed the same literature and reached more optimistic conclusions (Linetzky et al., 2015; MacLeod & Grafton, 2016).
In the addiction field, there have been promising results from a number of RCTs of one form of CBM (approach bias modification; Wiers et al., 2011, Manning et al., 2016; see this recent Mental Elf blog), although some researchers (including me and my colleagues!) have been skeptical about other forms of CBM (attentional bias modification; Christiansen et al., 2015). A meta-analysis of CBM for addictions would be a welcome addition to the field.
I first found out about the current meta-analysis over a year ago when I took part in a Mental Elf Campfire alongside the lead author (Ioana Cristea) and other colleagues who work on this topic. Ioana discussed her findings around the virtual campfire, so I was looking forward to seeing the article in print. The paper was published in PloS One in September 2016 (Cristea, Kok, & Cuijpers, 2016).
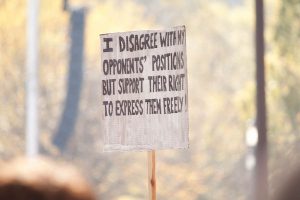
There are considerable levels of disagreement about CBM amongst researchers and practitioners.
Methods
The authors performed a comprehensive literature search to identify “randomised controlled trials” (RCTs; see Discussion) that investigated the effects of cognitive bias modification (a single session or multiple sessions) on cognitive biases and a range of addiction-related outcomes including subjective craving and clinician- or self-reported substance use, in comparison to any type of control condition.
They opted to exclude laboratory behavioural measures of alcohol consumption such as bogus ‘taste tests’ from their outcome measures, even though these were identified as primary outcome measures in many of the original studies (see Discussion).
For each comparison between a CBM and control intervention(s), effect sizes (Hedges’ g) were calculated at post-test and at follow-up. Data were analysed using a random effects meta-analysis. The number needed to treat (NNT) was also reported.
Publication bias was assessed using visual inspection of funnel plots accompanied by Egger’s test of asymmetry, and asymmetry was corrected with the Duval-Tweedie trim-and-fill procedure.
Twenty-five RCTs, from 24 published studies, were included in the meta-analysis. Study characteristics can be broken down as follows:
- 18 studies focused on alcohol problems, and 7 on tobacco smoking
- 12 studies targeted attentional bias, 8 approach bias, 4 response inhibition, and 1 interpretive bias
- 11 studies investigated effects of a single session of CBM, and 14 assessed the effects of multiple sessions (ranging from 2 to 21)
- Only 5 studies focused on patients who had been diagnosed with a substance use disorder (SUD); the majority (20) considered ‘consumers’ of those substances (e.g. students who consumed alcohol), in whom SUD status was unknown
- The majority of CBM interventions were delivered in a University laboratory (15), five in a clinical setting, and five in naturalistic settings such as participants’ own homes
- Seven studies included a follow-up, the duration of which ranged from one month to one year.
Results
- Effects of CBM (vs. control) on all outcomes at post-test (soon after receiving the intervention) were small and not statistically significant: g = 0.08 (95% CI = -0.02 to 0.18; NNT = 21.74). Heterogeneity was low (I2= 0%). Results were comparable for alcohol and tobacco outcomes, and when multiple comparisons from the same studies were considered separately
- There was no significant effect of CBM on post-test craving: 18 trials; g = 0.05 (95% CI = -.006 to 0.16; NNT = 35.71). However, the effect on cognitive bias at post-test was statistically significant: 19 trials; g = 0.60 (95% CI = 0.39 to 0.79)
- At follow-up, the effect of CBM on all outcomes was small but statistically significant: 7 studies; g = 0.18 (95% CI = 0.03 to 0.32)
- Subgroup and meta-regression analyses revealed that effects of CBM were not moderated by addiction type (alcohol vs. smoking), delivery setting (lab, clinic or naturalistic), CBM type (attentional bias, approach bias, response inhibition, or interpretive bias), sample type (patients vs. consumers). The number of CBM sessions did not moderate effects on subjective craving or behavioural outcomes, but it had unexpected and counterintuitive effects on cognitive bias outcomes: these effects were larger after a single session of CBM: 9 studies; g = 0.86 (95% CI = 0.53 to 1.18) compared to after multiple sessions: g = 0.35 (95% CI = 0.16 to 0.53)
- Most studies had high or uncertain risk of bias for most criteria; only 4 (of 25) studies had low risk of bias for at least three of the five criteria that were considered. A meta-regression revealed that risk of bias was negatively correlated with effect sizes for addiction outcomes: b = -0.11 (95% CI = -0.21 to 0.01), craving outcomes: b = -0.17 (95% CI = -0.29 to -0.06), but not cognitive bias outcomes
- The magnitude of the effect of CBM on cognitive bias did not predict the effect of CBM on addiction outcomes: b = 0.18 (95% CI = -0.07 to 0.44)
- There was evidence of publication bias, and adjustment for missing studies reduced the estimated effect size of CBM (on all outcomes at post-test) still further.
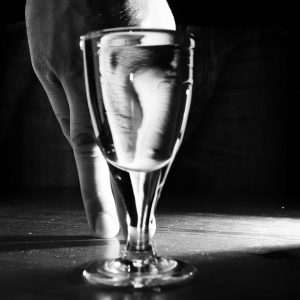
At post-test, there was no significant effect of CBM for addiction or craving, but there was a significant, moderate effect on cognitive bias.
Discussion
At first glance, these findings are very bad news for proponents of CBM. There was no appreciable difference between CBM and control conditions on all addiction outcomes at post-test. There was a small (but robust) effect of CBM on addiction outcomes at follow-up; however, most studies were estimated to have high risk of bias, and the extent of this risk of bias was positively correlated with the effect size: studies that had the highest risk of bias tended to yield the largest effect sizes. There was no evidence to suggest that some forms of CBM (e.g. approach bias modification) were more effective than others (e.g. attentional bias modification).
However, some colleagues and I have raised concerns about methodological issues with this meta-analysis that complicate interpretation of their findings (see this comment from myself, Paul Christiansen and Andy Jones (all University of Liverpool) and a separate comment from Reinout Wiers (University of Amsterdam). I won’t repeat these detailed observations here (I suspect that most readers will not be that interested!), but I will briefly discuss some of the main issues, the response from the lead author to these issues, and some insights from others after the inevitable discussion on Twitter that followed.
What constitutes a randomised controlled trial?
The first point is that 11 of the 25 studies included in the meta-analysis were psychology experiments that investigated the causal influence of cognitive bias on substance use or craving in the laboratory. They were never intended as ‘RCTs’, were not portrayed as such in the original papers, and initially we could not understand why Cristea et al. took the decision to treat them as if they were RCTs. True, the techniques used during CBM may be very similar, if not identical, to experimental tools that were designed to manipulate cognitive bias in psychology experiments, but does this mean that both types of research should be described as RCTs? The authors subsequently responded to our comment and pointed out that according to both NICE and Cochrane guidelines, ‘RCTs are identified as such by the existence of a random allocation of participants to two or more groups, with one receiving an intervention, and the other no intervention, a dummy treatment or no treatment’. They went on to state that to distinguish laboratory studies from RCTs would be ‘cherry picking’.
So, do we stand corrected? I’m still not convinced, and I think that it hinges on how one defines an ‘intervention’. The implication of such a broad definition (as applied by Cristea and colleagues) is that any psychology experiment which compares the effects of two experimental manipulations on clinically-relevant outcome measures (e.g. substance craving or consumption, or subjective mood in the case of emotional disorders) should be classified as an RCT. I do not agree. However, I accept the point that we should not deliberately omit potentially valuable data from meta-analyses simply because those data were not labeled as an RCT in the original papers.
This issue provoked some polarised responses on Twitter, and it could rumble on indefinitely. However, it may all be irrelevant because Cristea et al. did investigate whether single-session lab studies (usually with student volunteers) and multiple-session CBM studies (often, but not always, with substance-dependent patients) yielded different findings: they did not. However, this failure to appreciate the difference between laboratory research and RCTs may have had other consequences, as detailed below.
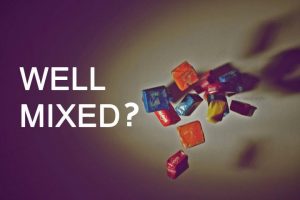
Should laboratory-based psychology experiments be included alongside RCTs in meta-analyses like this one?
Why disregard data from laboratory measures of alcohol consumption, such as the bogus ‘taste test’?
The second point concerns the outcome measures from laboratory studies of alcohol CBM that were selected or excluded from the meta-analysis. In many of these studies, participants completed a bogus ‘taste test’ immediately after completing the CBM (or control) intervention. During the bogus taste test, participants were given alcoholic drinks (often alongside soft drinks) and instructed to drink as much or as little as they wished in order to rate the taste of the drinks. Despite these instructions, the real purpose of bogus taste tests is to record how much alcohol participants choose to drink, the volume of which can be standardised across studies (by expressing it as a percentage of the volume of alcohol provided or, if participants have a choice between alcoholic and soft drinks, by expressing alcohol intake as a percentage of total fluid intake). This task is widely used in laboratory research on addiction, and comparable tasks have been used to investigate influences on food intake in the laboratory for many decades (see Jones et al., 2016, for our recent paper on the construct validity of the task). However, Cristea et al. opted to exclude all outcome measures from bogus taste tests from their meta-analysis because ‘this is a non-standardized and variable task that does not take into account participants’ general preferences, their habitual alcohol consumption, and that is usually carried out without their awareness’.
In our commentary, we noted our objections to the decision to exclude outcome variables from bogus taste tests. One observation is that each of the objections identified by Cristea et al. (in the above quote) are also likely to apply to subjective craving and self-reported alcohol consumption, the outcome measures that Cristea et al. chose to include. For example, subjective craving questionnaires differ widely from each other (in terms of their factor structure etc.); therefore, why are these any more ‘standardized’ than bogus taste tests? Also, participants who normally drink heavily and prefer alcoholic drinks over soft drinks are also likely to report higher craving for alcohol; so subjective craving would also be influenced by their ‘general preferences and their habitual alcohol consumption’. Finally, in my opinion the fact that participants are deceived about the real outcome measure when they complete ‘bogus’ taste tests is a methodological strength, rather than a weakness. Perhaps a more important, overarching point is that none of these methodological limitations should really matter if participants are randomly allocated to experimental conditions, so long as the same outcome measures are measured in all participants. Which of course, they are, in both psychology experiments and RCTs (back to this distinction again).
I would like to see this meta-analysis repeated with inclusion of standardised outcome measures from bogus taste tests. For the record, I don’t anticipate this changing the overall conclusions!
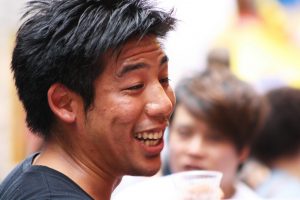
Would this evidence be more reliable if bogus taste test outcomes were included in the analysis?
Are risk of bias measures appropriate for psychology experiments?
In a separate commentary, Reinout Wiers identified additional methodological limitations with this meta-analysis. One problem is the way that ‘risk of bias’ was assessed, which again goes back to the failure to distinguish between psychology experiments and RCTs. The issue is that some of the risk of bias measures (e.g. failure to report the method of randomisation, to report dropouts or confirm that intention to treat analyses were used) simply do not apply to psychology experiments. This is because randomisation is determined by a random number generator or is automated (e.g. by the computer program that ‘delivers’ CBM) and student volunteers typically do not drop out of brief psychology experiments, so the dropout rate is often zero (in which case, it is not reported). Therefore, the risk of bias estimates are likely to be inflated because criteria intended for RCTs were applied to psychology experiments for which these criteria were never intended.
Conclusions
The overall conclusions from this meta-analysis are a much-needed tonic to the (often uncritical) wave of enthusiasm for CBM for addiction and other psychological disorders. Unfortunately, in my opinion, the authors’ criterion for what constitutes an RCT is far too broad, and they fail to recognise the distinction between psychology experiments that manipulate candidate psychological processes in order to investigate their influence on subjective craving and substance use, versus RCTs that evaluate the effectiveness of psychological interventions for substance use disorders. The consequences include application of risk of bias measures and unwarranted exclusion of a key outcome measure. Overall, I believe that this paper does not add much clarity to the literature on CBM for addiction, which is a shame.
To end on a conciliatory note: how are people who do not work in the CBM field supposed to know the difference between a psychology experiment and an RCT? Perhaps what is needed is a framework that enables us to clearly distinguish psychology experiments and experimental medicine from ‘genuine’ RCTs, to develop appropriate risk of bias assessments for these different types of research, and to pay closer attention to the reliability and validity of the outcome measures that are used in these different types of research.
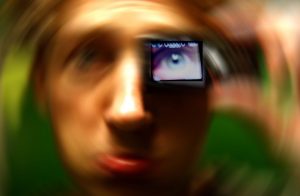
We need a framework that helps distinguish psychology experiments and experimental medicine from ‘genuine’ RCTs.
Conflict of interest
I work in this field, and several studies from our group (some with positive findings, some with null results) were included in the meta-analysis. I admit that I wish that CBM would prove to be an effective intervention for substance use disorders, but I do not consider this to be a conflict of interest and, as noted in the Introduction, I have previously expressed my skepticism about some forms of CBM in print.
Acknowledgements
I am particularly grateful to Reinout Wiers, Andy Jones, and Paul Christiansen for useful discussions when preparing comments on this article for PLoS One, and to Marcus Munafo and Anne Wil-Kruijt for their insights when the discussion continued on social media.
Links
Primary paper
Cristea IA, Kok RN, Cuijpers P (2016) The Effectiveness of Cognitive Bias Modification Interventions for Substance Addictions: A Meta-Analysis. PLoS ONE 11(9): e0162226. doi: 10.1371/journal.pone.0162226
Other references
Christiansen, P., Schoenmakers, T. M. & Field, M. (2015). Less than meets the eye: Reappraising the clinical relevance of attentional bias in addiction (PDF). Addictive Behaviors 44, 43-50.
Cristea I. A., Kok, R. N, & Cuijpers, P. (2015). Efficacy of cognitive bias modification interventions in anxiety and depression: Meta-analysis. British Journal of Psychiatry, 206: 7–16. [Mental Elf blog of this study]
Jones, A., Button, E., Rose, A. K., Robinson, E., Christiansen, P., Di Lemma, L., et al. (2016). The ad-libitum alcohol “taste test”: secondary analyses of potential confounds and construct validity. Psychopharmacology, 233: 917–924.
Linetzky, M., Pergamin-Hight, L., Pine, D. S., & Bar-Haim, Y. (2015). Quantitative evaluation of the clinical efficacy of attention bias modification treatment for anxiety disorders. Depression and Anxiety,32: 383–391. [PubMed abstract]
MacLeod, C., & Grafton, B. (2016). Anxiety-linked attentional bias and its modification: Illustrating the importance of distinguishing processes and procedures in experimental psychopathology research. Behaviour Research and Therapy, doi:10.1016/j.brat.2016.07.005. [Abstract]
Cognitive bias modification for addiction
Are we flogging a dead horse?
https://t.co/mnD7elTtQ9 https://t.co/iDkGBxPd0L
Cognitive bias modification for addiction: are we flogging a dead horse? https://t.co/SCPyFHsEYa
Today @field_matt on CBM for substance addictions
New @PLOSONE meta-analysis by @Zia_Julia @pimcuijpers… https://t.co/6Rnsbiw7Rp
Cognitive bias modification for addiction
Are we flogging a dead horse?
https://t.co/mnD7elTtQ9 https://t.co/KnuZeZN94J
It would have been useful to actually read the risk of bias section. No drop-outs post randomization (drop-outs by the way were not so unlikely as it “would seem reasonable to assume”) were rated as low risk of bias. So the entire objection about inflation of risk of bias ratings is moot. If anything, every criterion was flexed so as to make it more attainable for psychological interventions. So maybe prof. Wiers should actually read the method section (strange, given he was also a reviewer). Not only this, but individual ratings for each study are given in the Appendix. It’s an easy check. The part about randomization I don’t understand. If there was ANY mention of a method using a random element, including drawing lots, this was coded as low risk of bias. Evidently this referred to assignment to groups, not to how participants in a group got the stimuli. Again unless the logic is “studies used a computer, hence everything must be ok”, in which case I say we all go home. So either the Cochrane is a projective test (in many ways maybe it is), even the extensive risk of bias section was not really read, but both critiques about risk of bias being inflated are just mystifications.
I read the risk of bias section, including the appendix, carefully before writing the blog. Thanks for giving me the opportunity to clarify that. Unfortunately I did not have space in the blog to go into this issue in detail, and in retrospect perhaps I should have. I think it comes down to whether you think that psychology experiments and RCTs are the same, and therefore whether it is appropriate to apply the same risk of bias assessments to both. Cristea and colleagues have made their position clear, as have I. Perhaps, for now, we just have to agree to disagree or, if we can’t do that, agree that we need a better framework to clarify the relationship between lab research, experimental medicine, and RCTs, and to determine appropriate risk of bias measurements for these different types of research (if we can ever agree that they are indeed ‘different’).
Even though I did not discuss this issue in detail, I did direct readers to a detailed comment from Reinout Wiers that discussed the issues with risk of bias assessments for lab studies in some detail (http://journals.plos.org/plosone/article/comment?id=info%3Adoi/10.1371/annotation/8cde1186-7802-4dd5-b686-d4a66c84fb84). Interested readers might like to take a look at this, and perhaps Cristea and colleagues could respond to those detailed points (either here on the Mental Elf, or on the PloS site).
On a related point about the association between risk of bias and effect size, I did not understand why the authors computed a ‘low risk of bias’ score for this analysis, rather than just using ‘risk of bias’ (which should be a linear scale, after all) and using that for this analysis. I would be interested to know what this alternative analysis throws up – are the findings the same? (i.e. is risk of bias positively correlated with effect size?) If the response is that this is not possible because risk of bias was ‘unclear’ for too many criteria for too many of the lab studies (which it appears to be, according to the table in the appendix that I apparently have not read), then maybe this tells you something about how appropriate those risk of bias criteria were in this case.
Finally, I went to some lengths to point out that I am skeptical of CBM for addiction (read conflict of interest statement at the end of the blog). I might also point out that I have done several lab studies on CBM (which I feel have been misrepresented here), but to date have not been involved in a single published trial. So it’s a bit irritating (but predictable) to be called out for ‘defensiveness and distortion from reviewer whose work criticized’ as as part of the ‘establishment’ on social media, just because I disagree with the methods used.
I’m sorry, this explanation is just not on the point. I appreciate you read (I am sure of that really), but this was the most innocent possible explanation. Other than that you present two inaccuracies about the way we assessed risk of bias. I don’t see how that is something debateable. That R. Wiers, the Pope or Santa Claus said them in the first place is just irrelevant. What matters is they are not true and by choosing to include them you maintain this impression that CBM studies were whipped with incredibly elaborated whiplash or held at these impossible standards. It was not, quite the contrary. The standards were adapted, even lowered, and guess what? We clearly stated how we rated each criteria of risk of bias. If there were no drop-outs (considered from all randomized participants), we coded low (for readers, that means good). This by the way is in no means the norm, usually not talking about drop-outs or just saying they were few warrants a rating of uncertain. The position you mention (“students! how could they possibly drop-out”) is not only simplistic (in some cases some people were excluded post-randomization, because well, the experimenter felt like they were not compliant. true story) but it actually speaks of the complete lack of accountability of this field. You don’t even seem to fathom the idea that drop-outs might be relevant because they might point to problems in the intervention. Imagine is a biotech scientist just testing a “substance” or device in “laboratory” studies first and not even mentioning this. We would all be outraged. But then when experimental psychopathology studies do it, it’s just regular practice. All in all, I have no issue with your blog, one is entitled to comments and that is a conversation. I do have a big issue with including inaccuracies particularly for information that is amply there.
Please do not attribute James Coyne’s comments to me. And also a declaration of COI that includes the statement “I don’t think I have COI”… well there is some irony there. Regarding responding to comments to Plos One, I think it should be appreciated 1. they are simply reader comments so that is a bit different (there is no actual peer review involved) and I already took the time to respond to one and 2. it’s almost hilarious to imply that I don’t respond to one comment because I am not sure what to say. Prof. Wiers chooses to do a sweeping rant of ALL our meta-analyses on CBM (forgetting not only that they passed through rigorous peer review) in a post rife with the same inaccuracies and/or doubtful arguments we already addressed and he does not seem to see even a small problem with the fact that he is actually the intervention developer and author of most trials. I think that disqualifies the comment.
On why we did not use a risk of bias score. We did. Linearly, exactly as we say. We called it low risk of bias for the reader to understand high scores on it are a good thing. But to make it linear (risk of bias is coded categorically), as we CLEARLY state in the methods section, we gave 1 point for each criteria that had low risk of bias (“good”), 0 for all the rest. I don’t see what the alternative would have been. Give 1 point for high or unclear risk of bias? Where is the confusion? It is an exploratory analysis we did not overinterpret. And a relationship that was shown not only in MANY psychotherapy meta-analyses, but- surprise, surprise- in quite a few meta-analyses of CBM interventions.
Also, MacLeod & Grafton is simply a narrative review and should not be referred to as a meta-analysis. I hope we don’t start to perpetuate the myth is actually a meta-analysis; it factually isn’t. This also means it’s an idiosyncratic interpretation of the literature, in no way on par with evidence from meta-analyses. Other uncited meta-analyses also found negative effects of CBM (Heeren et al., 2016; our own meta-analysis on children and adolescents). And negative results RCTs are flocking. So just that we keep the counts right, the situation is FAR from being “promising but slightly controversial”.
Fair points – and I apologize for inadvertently misrepresenting the MacLeod & Grafton paper, which was an important precursor for the Linetsky et al. paper that followed
The Linetzky meta-analysis is a perfect example of a poorly conducted MA tailored by authors with massive researcher allegiance to promote positive results based on underpowered and methodologically weak studies. Perhaps I should do a re-analysis of the data set but I can’t be bothered to input the data and I’m sure Linetzky will conveniently have ‘misplaced’ the CMA data file or some other guff.
Two corrections to the statements made above. 1. I was not a reviewer of the CBM Addiction paper. When it was submitted to the journal addiction, the editorial team decided to send it to two anonymous reviewers, one critical of CBM, and the other more positive about CBM, who agreed that it was inappropriate to combine experimental lab studies and clinical trials. I agree with Matt that that is the heart of the matter.
2. MacLeod and Grafton is indeed not a meta-analysis, but does report the re-analysis of the Cristea et al 2015 meta-analysis which has been mysteriously held up for publication for over a year now by an anonymous reviewer. And fact of the matter is that there is no effect on psychopathology symptoms in those studies that did not succeed in changing the bias and there is a medium sized effect in those studies that did change the bias.
We publish meta-analysis w/ negative results, establishment goes crazy. Some errors in blog https://t.co/rc2N7ztOUD @Mental_Elf @robinnkok
#CBM for #addiction
Robust response from @Zia_Julia
To critique by @field_matt
Join the discussion!… https://t.co/hTxBGO0vxC
Meta-analysis finds no significant effect of cognitive bias modification #CBM for addiction or craving… https://t.co/IHJJM94bOs
Cognitive bias modification for #addiction: are we flogging a dead horse? https://t.co/pKauldCTES @Mental_Elf on a flawed #metaanalysis #CBM
@NHFTNHSLibrary Wow, flawed, really? As the author I could not disagree more. I wish you would point the flawed parts.
RT @Mental_Elf: Meta-analysis finds no significant effect of cognitive bias modification for addiction or craving https://t.co/D57g9AyUQ6
@field_matt On recent CBM meta-analysis for #addiction. A lot of debate… great lunchtime reading https://t.co/k4te8biVek @Mental_Elf
https://t.co/ItoQw84ive
Should lab-based psychology experiments be included alongside RCTs in meta-analyses on cognitive bias modification?… https://t.co/gTD7sdYfXT
RT @Mental_Elf: Cognitive bias modification for addiction
Are we flogging a dead horse? https://t.co/D57g9AyUQ6 https://t.co/0eLS09A5gm
On the issue of behavioral outcome measures (the “taste test”), we already responded at length. So since none of this made its way into the post, I will re-copy the reply from the Plos One website here.
“The second main critique regards our choice of outcome measures. Field and colleagues criticize us for not including alcohol consumption as measured by the “taste taste”. As it is clearly stated in our paper, we did include alcohol consumption or other measures of substance consumption (like cigarette consumption) when they were measured with a standardized instrument (such as the Alcohol Approach Avoidance Questionnaire/AAAQ, in fact developed by one of the CBM developers, or The Timeline Follow-Back diary/TLFB). We did indeed not consider behavioral measures such as the taste test. This task measures in the laboratory how much the participants drink from an alcoholic (usually beer) and respectively non-alcoholic drink (usually orange juice). We were unable to find any independent validation of this measure, particularly regarding its criterion validity. Field and co-authors claim to have demonstrated a correlation between how much participants drink in the lab and how much they drink in the laboratory. The paper they cite in the comment is their own meta-analysis showing that a form of CBM has a positive impact on food or alcohol consumption measured in the laboratory. We were not able to find the result they made reference to in that cited paper. However, in another paper, unfortunately not referenced in the comment[3] Jones and colleagues did show, as claimed in the commentary, that participants’ typical alcohol consumption was a significant predictor for their performance on the taste test. However, in the comment Field et al. omitted to mentioning other relevant results from this paper. For instance, typical alcohol consumption was a significant predictor together (i.e., in the same model) with subjective craving and the perceived pleasantness of the alcoholic drink. All these three variables were related to consumption as measured by the taste test. Also, hazardous drinking as measured by the Alcohol Use Disorders Identification Test (AUDIT) was not related to consumption as measured by the taste test. Hence, this is hardly evidence of validity. We still don’t know which one of these variables is more important in accounting for the performance in the taste test. Maybe it’s subjective preference, which would not really be related to addiction (unless of course to like beer more than orange juice is a problem). In fact, what appears evident from Jones at al. [3] is that it is not clear what behavior at the taste test means and that this behavior is most likely connected to a number of factors. Importantly, social desirability, which could have been very relevant given the nature of the problem and the study population (mostly young females) was not considered. Nonetheless, there is an even more problematic aspect in considering this paper as evidence of criterion validity. The analysis presented was not carried on an independent sample, but aggregating data from separate intervention studies. Jones et al.[3] claim to have demonstrated the construct validity of the taste test because they showed it is sensitive to experimental manipulations (mainly several forms of CBM). But this argument is circular. The authors are doing what is known as a “double-dip” in their evidence. On one hand, they use these studies as separate studies proving the efficiency of CBM interventions because of a demonstrated effect on participants’ performance in the taste test (in the single papers and in their meta-analysis[4]). On the other hand, they collate a very similar pool of studies (some are identical) to show these prove the validity of the taste test as a relevant outcome measure because it was successfully impacted by CBM interventions. A proper validation analysis would require a separate, new sample. Moreover, we would add that proper establishing of criterion validity for the taste test should not simply show that it correlates with consumption, this is of course evident. If you don’t drink beer, you will not drink it in the laboratory. Rather, validation ought to show that this behavioral measure is sensitive enough to detect relevant variations in drinking behavior, i.e., if you drink more outside the laboratory, you would tend to drink more in the taste test.
In sum, we are perplexed as to why Field and colleagues find our decision to exclude ad hoc non-validated behavioral measures confusing or arbitrary. They argue this decision was not supported by any references but this because there were no references validating the taste test as a substitute measure of addiction relevant outcomes. As we explained previously, citing the authors’ own research, it is not even clear what the taste test measures. Does it measure consumption? Does it measure preference? Does it measure the fact one simply does not like orange juice very much? As we are talking about a sensitive behavior like alcohol consumption, particularly given that a significant proportion of participants were aware of the purpose of the taste test, it would also be reasonable to question how this measure fares in regards to social desirability. Nonetheless, a more serious problem for the taste test in regard to our meta-analysis was that studies used different versions and very different scoring procedures. This is not simply a trivial problem to be just noted as a limitation, it cannot be simply filed under “the measure is not perfect” as Field and colleagues seem to suggest in their comment. This is a major issue particularly germane for a meta-analysis as it would have led to very high heterogeneity around the estimations of the effect. This means that the pooled effect size could have no longer been considered a reliable estimation of the “true” effect of the intervention. It also did not help that outcomes on the taste test are given in ml, a unit that simply cannot be aggregated with measures such as psychological scales. We do not have any standardization for this measure, so as to be able to tell how many ml of beer (or orange juice) drank in the lab are a little or a lot. As importantly, any standardization should probably take into account participant’s baseline values.
Field and colleagues also criticize us for considering craving as a more important outcome and seem to imply we did not take into account the existent limitations of measures of craving. This is inaccurate. Craving was simply a construct that was assessed in more trials and as such we could aggregate results. Some of these studies used validated scales, some used visual analogue or Likert scales. As evident from Table 2, we conducted sensitivity analysis exclusively examining the validated measures of craving and results were similar. We believe this is evidence we did acknowledge the limitations of the craving measures since we thought it necessary to verify them in sensitivity analysis. Moreover, we did not use attempt to speculate on the meaning of these results for addiction research, and simply reported them because craving was a relevant outcome measured in a sufficient number of studies. Surely, Field et al. can see that there are considerable differences between interpreting a construct like urge to drink, even when measured on a Likert scale, and interpreting the quantity of beer consumed in the laboratory, or in many cases an obscure proportion between the quantity of beer consumed and that of orange juice, as done by variations of the taste test.”
And as I conclude in that comment and I will state again, we are more than willing to recognize that if synthesizing the main critiques, the claim would be that while CBM might not work for addictions, it could be effective on making an individual drink orange juice or soda rather than beer in a laboratory setting, that might indeed be true.
“Cognitive bias modification for addiction: are we flogging a dead horse?”
Yes
@phillips_jacks https://t.co/SIvvj7mTZY
Cognitive bias modification for addiction: are we flogging a dead horse? https://t.co/G53emvRHxv
Don’t miss:
Cognitive bias modification for addiction
Are we flogging a dead horse?
https://t.co/mnD7elTtQ9 https://t.co/wZUKq0el39
Cognitive bias modification for addiction: are we flogging a dead horse? https://t.co/OGFgALvdDh via @sharethis
Cognitive bias modification for addiction: are we flogging a dead horse? https://t.co/3hZhYEipJb via @sharethis
Cognitive bias modification for addiction: are we flogging a dead horse? https://t.co/8CJFDMH6Xo https://t.co/FpgYHy4d0w
[…] to the object of that addiction. It seeks ways to help people process information differently. This article looks at a meta-analysis of studies into the effectiveness of Cognitive Bias Modification. The […]