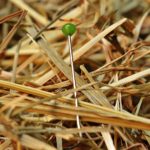
Mental health services are partly focused on preventing bad outcomes (Jankins, 2014). Major adverse outcomes in mental health services include worsening mental health, suicide, and violence towards other people, for example.
Professionals working in mental health services, but also social care, general health services, criminal justice, and many other public services, often need to make decisions based on some evaluation of the likelihood of future bad outcomes, such as suicide or physical violence. This process, risk assessment, is widespread, and often involves the professional completing structured tools (often in the format of a questionnaire), and on this basis classifying the person into levels of future risk.
A professional who wishes to carry out a risk assessment, e.g. for future suicide risk, will sometimes select from a list of available tools and select an appropriate tool. Tools often require some specific training, and a dedicated meeting with the service user in order to complete this. More often, professionals generate unstructured assessments of risk, based on their professional judgement.
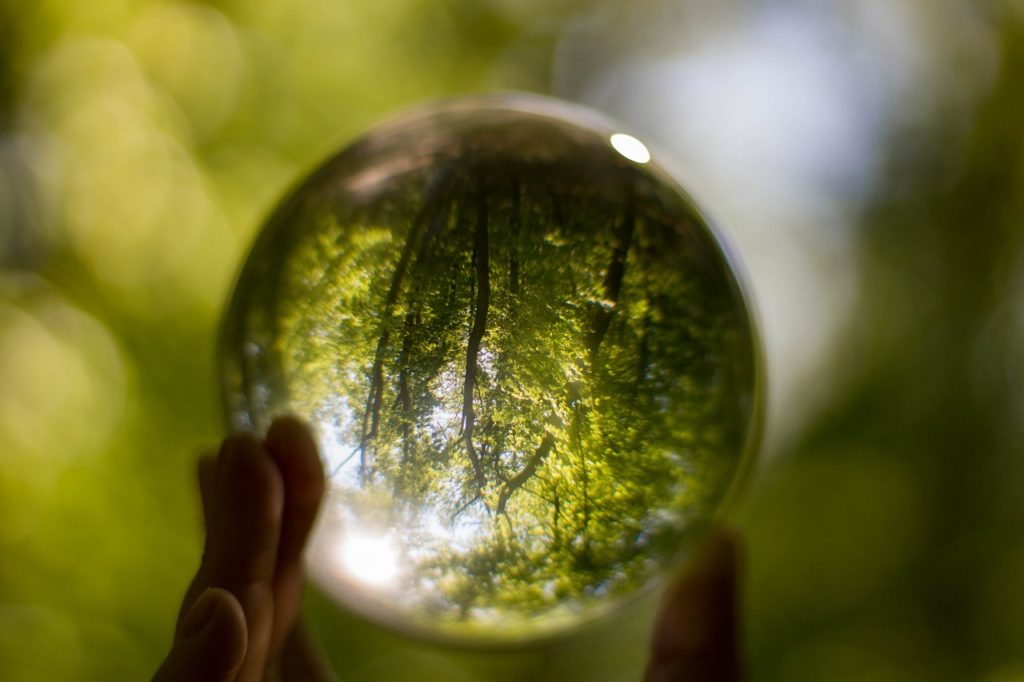
Many bad outcomes such as violent crime and suicide are rare, so predicting when they are likely to happen is extremely challenging.
The effectiveness of risk assessment
Ultimately the quality of risk assessment processes is driven by the frequency of adverse outcomes. Given that many bad outcomes, including violent crime and suicide, are rare, prediction is extremely challenging. This crucial challenge has been taken up by statistical methods that aim to summarise previous data on what factors predict a particular outcome, so that clinicians can meet the next service user and make an accurate assessment of future outcomes (“clinical prediction”). The idea is that this information can therefore be used to guide later management.
Although sometimes controversial, these statistically informed risk assessment tools have been proposed as complementary to usual clinical care. Risk assessment is part of routine mental health practice, it is argued, and tools based on data, if used appropriately, can be important aids to decision-making.
Currently used risk assessment tools are labour intensive, expensive and impractical for wide scale implementation, for example in generic mental health services. Few existing risk assessment tools are empirically derived and validated; in other words, it is impossible to work out, for most tools, how the variables included in the tools were selected, and how decisions were made on the number of items to include in the tool, or how accurate the tool is in predicting the relevant outcome (Fazel et al, 2018). Nevertheless, well performing tools may be useful indicators of baseline risk, and as a way of identifying higher risk individuals.
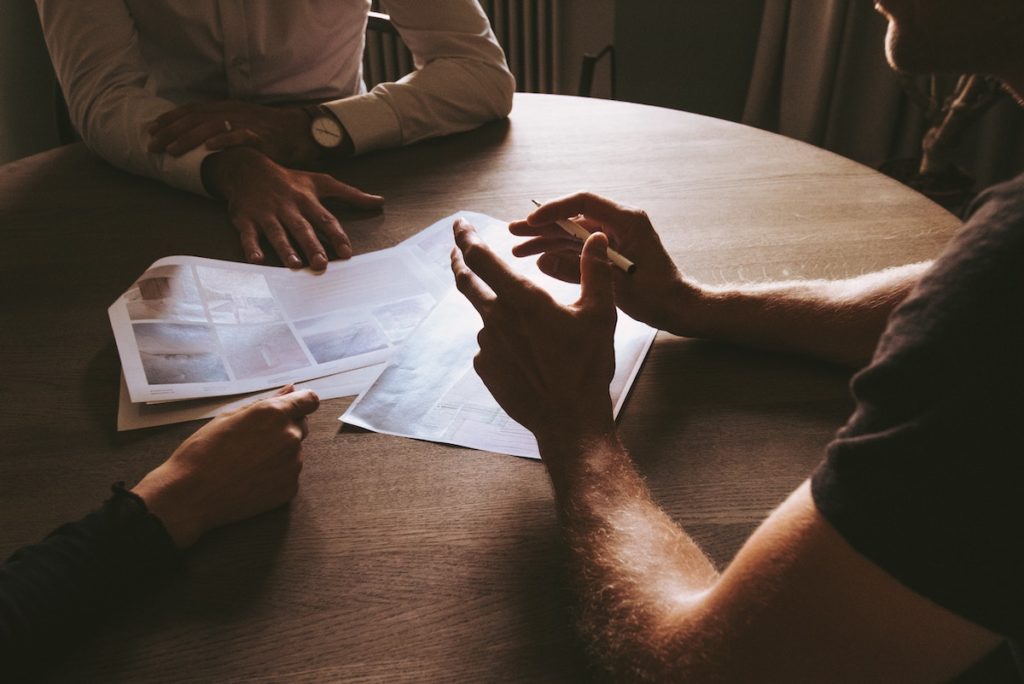
Although sometimes controversial, statistically informed clinical prediction tools have been proposed as complementary to usual clinical care.
Methods
Fazel et al’s paper (2019) reports the development of a clinical tool to predict suicide in people with severe mental illness: the OxMIS (Oxford Mental Illness and Suicide) tool.
Clinical prediction tools aim to summarise what is known on the risk factors for a particular outcome in a particular population, so that this information (in the form of weights applied to certain risk factors) can be used to guide clinical decision-making. Therefore, deriving a prediction tool involves studying the occurrence of the outcome you are interested in, in this case suicide, in the population in which you want to implement the tool, in this case people with severe mental illness.
The investigators wrote down what they were going to do before they started the study. In this study, the authors used Swedish national registers to follow up all Swedish individuals aged 15-65 with a diagnosis of severe mental illness, which included schizophrenia and bipolar disorders, with diagnoses made between 1 Jan 2000 and 31 Dec 2008, for episodes of admission. They identified 75,158 patients, in whom there were 574,018 admissions. For each patient they selected one admission at random. Each individual was followed up from the day of discharge from that admission, for one year. The outcome for prediction was suicide, which incorporated deaths coded as suicide according to the 10th edition of the WHO international classification of disease (ICD-10), and deaths that were coded as undetermined. Information on risk factors for suicide was taken from other administrative registers in Sweden, including information on sociodemographic risk factors, medication, and previous violence conviction. The authors developed a statistical model for suicide in this cohort, ignoring deaths from other causes, and ignoring emigration from Sweden during the study period.
In order to select which variables to include in the final tool (see table 1 below), all the risk factors were grouped into the presumed strength of the association with suicide. They left out any variables where more than a third of admission had missing data. For the other variables, missing data was “filled in” based on assumptions about the reasons for data being missing, a method called multiple imputation. In multiple imputation, you fill in missing values based on what you know about the values of the variable that is missing, based on the data that are present, and use this model to predict the missing values, creating an imputed dataset. A number of imputed datasets are created, combined, and then the main model is derived based on this combined data.
Predictor variables included in the OxMIS (Oxford Mental Illness and Suicide) tool
Table 1 | ||
---|---|---|
Variable | Odds ratio [95% CI] | p-Value |
Sex: male | 1.92 [1.58 to 2.33] | <0.001 |
Age (per 10 years) | 0.92 [0.85 to 0.99] | 0.02 |
Previous violent crime | 0.78 [0.60 to 1.02] | 0.07 |
Previous drug use | 1.09 [0.84 to 1.41] | 0.54 |
Previous alcohol use | 1.29 [1.02 to 1.63] | 0.03 |
Previous self-harm | 2.55 [2.09 to 3.11] | <0.001 |
Educational level | ||
Upper secondary | 1.24 [1.00 to 1.53] | 0.05 |
Post-secondary | 1.68 [1.24 to 2.28] | <0.001 |
Parental drug or alcohol use | 0.70 [0.50 to 0.99] | 0.04 |
Parental suicide | 1.75 [1.14 to 2.69] | 0.01 |
Recent treatment: antipsychotic | 1.29 [0.98 to 1.69] | 0.07 |
Recent treatment: antidepressant | 1.75 [1.29 to 2.38] | <0.001 |
Inpatient at the time of assessment | 2.95 [2.45 to 3.55] | <0.001 |
Length of first inpatient stay > 7 days | 1.23 [1.00 to 1.50] | 0.05 |
Number of previous episodes > 7 | 0.77 [0.61 to 0.97] | 0.03 |
Benefit receipt | 0.83 [0.67 to 1.02] | 0.07 |
Parental psychiatric hospitalisation | 1.20 [0.97 to 1.48] | 0.10 |
Comorbid depression | 1.27 [1.03 to 1.56] | 0.03 |
Table 1 (above): A list of predictor variables included in the final tool. Each variable is accompanied by a number (an odds ratio) reflecting the strength of association with suicide, which can be considered a measure of how each variable is weighted in order to produce the final estimate of probability. With thanks to Fazel et al.
Prediction tools and how to make one
Prediction tools are usually based on a type of regression model. Regression models are summaries of the relationships between a series of variables and some sort of outcome of interest, based on data, and some assumptions about how the data came to be generated. When appropriately developed, these models summarise previous data on which variables predict and outcome, and how strongly, so that this information can produce an overall estimate of future risk. Therefore, the idea of a prediction tool is that at the point of carrying out the tool on a patient, relevant clinical and sociodemographic information could be entered into the equation, to estimate the risk of a future outcome.
Building the initial tool, based on data you already have, is the easy part. The next step is to test if the tool is accurate at predicting the outcome. This process is called validation, and is done in two steps (ideally).
- Firstly, the tool is tested to see if it accurately predicts the outcome in the same sample population from which the tool was derived, a step known as internal validation.
- After satisfactory internal validation, the tool is tested in new data, from a different sample population (external validation).
During internal and external validation, Fazel et al. used various accepted measures of accuracy. These measured reflected how good the tool was at distinguishing those who did and did not die of suicide, whether the tool was generally inaccurate in a consistent way, how well the tool reclassifies patients compared to other possible tools, and how often the tool was right (in either direction, i.e. at predicting suicide, and at predicting no suicide). The authors published the final tool online as a web calculator, for public use.
The investigators classified possible predictor variables into three groups, in order of presumed strength (basically, how well each variable was expected to predict suicide):
- The strongest group of predictors was entered first, to derive a “first go” tool.
- Then they added the second strongest group of predictors, keeping the ones that were statistically associated with the outcome, and discarding the others. Among the variables in this group, diagnosis was not statistically associated with suicide, and was taken out of the tool.
- Finally, to these variables, they added a final set of relatively weakly predictive variables, again leaving out those variables that were not statistically predictive of suicide. This left 17 variables in the final tool.
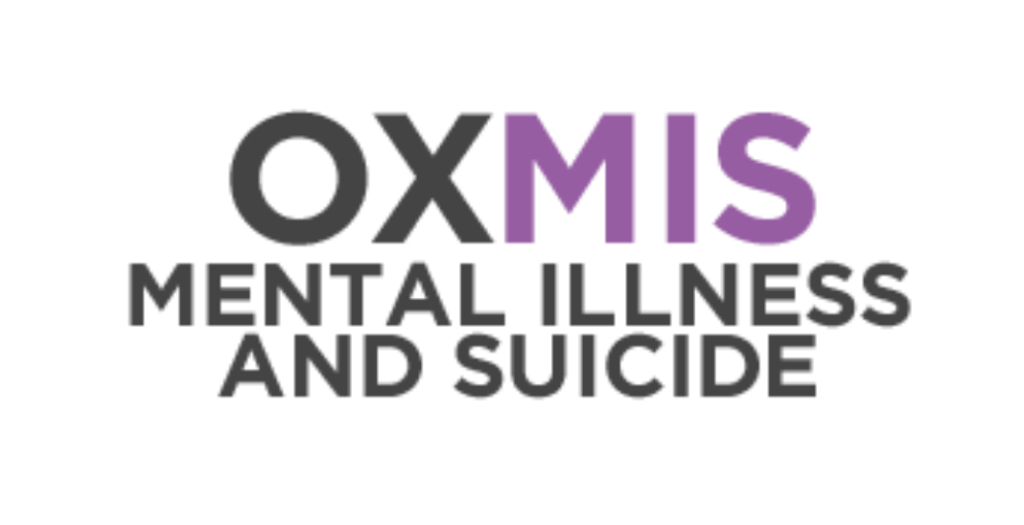
Try OxMIS out for yourself at: oxrisk.com/oxmis/
Results
Of around 75,000 people diagnosed with schizophrenia in this study, 59,000 were used as the basis for developing the prediction tool, and the remainder, around 16,000, for validation. There were 494 suicide deaths in the first group, and 139 deaths in the validation group. The strong predictors of suicide in this group were as expected:
- Being an inpatient at the time you were assessed was accompanied by a 3-fold increase in your odds of dying by suicide
- Those with previous self-harm experienced a 2-fold greater likelihood of dying by suicide
- Suicide was nearly twice as common in men than women
- Suicide became gradually less common with increasing age.
The final tool showed good discrimination; that is, it turned out that the tool was good at differentiating between higher and lower risk people. The investigators estimated that around three-quarters of people who died by suicide had higher scores, based on this tool, than those who did not, suggesting that the tool was good in this regard. Predicted and actual probabilities were very similar, also indicating that the tool was well calibrated. The tool achieved similar measures of performance in the derivation data and the validation data.
At this stage, the tool estimated the true probability of later suicide (i.e. a number ranging from 0 to 1). On the other hand, clinical decisions often depend on applying cut-offs for decision-making based on acceptable levels of risk, and this was investigated in the subsequent steps. The authors applied a cut-off of 1% risk of suicide in one year, finding that in these circumstances the tool was:
- not very sensitive (classifying true suicides correctly in 58% of everybody who died by suicide)
- not that specific either (classifying correctly people who did not die by suicide in 76% of people who did not die by suicide).
The study used routinely collected data to produce a tool for predicting suicide, including a list of variables (such as age, diagnosis, gender), with weights, used to derive an overall probability of later suicide. When comparing this tool with the actual observed data, the tool performed reasonably well. This is the first tool developed and externally validated to predict suicide in bipolar disorder and schizophrenia.
The OxMIS tool is presented as web-based calculator to give a personalised estimate of risk, to guide clinicians assessing suicide risk. The authors suggest that methods to derive a continuous measure of suicide risk could raise the overall quality of risk assessment, allow discussion between clinicians from different teams, and transparent communication on risk.
It is important to point out that the tool (and indeed, any tool of this type), incorrectly classifies a proportion of people who get the outcome, and therefore that the tool should not simply supersede usual clinical indicators. The tool could be seen as a cost effective summary of existing clinical knowledge, given that the tool is derived on data from a sample receiving interventions, and clinical assessments of risk. Such tools are also able to cope with uncertainty about the nature of some of the variables, such as employment status, or diagnosis.
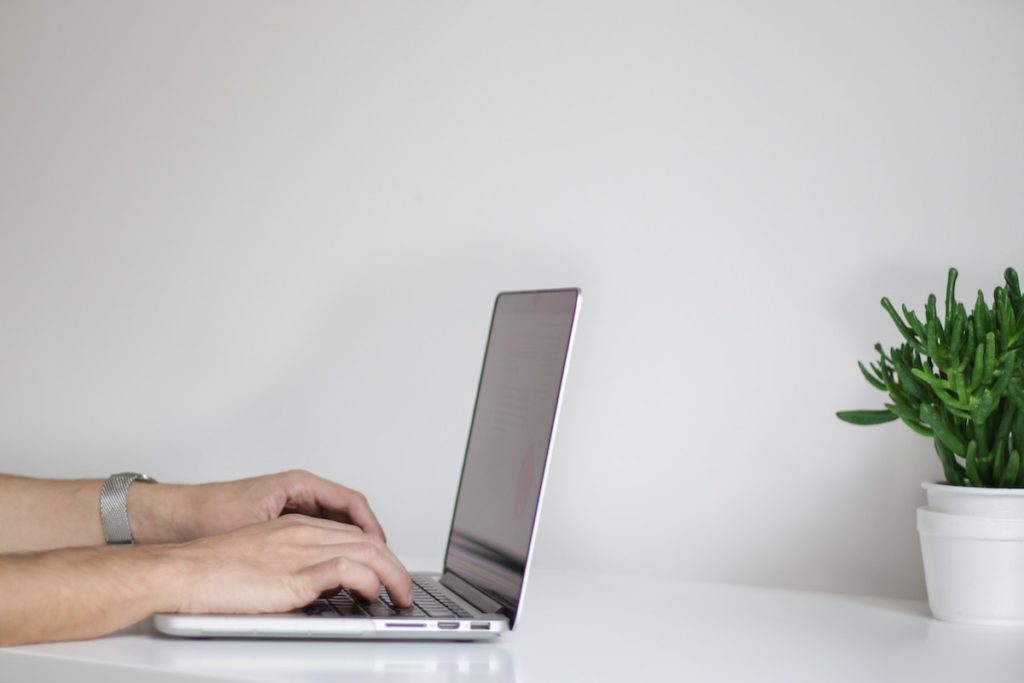
This is the first tool developed and externally validated to predict suicide in bipolar disorder and schizophrenia. The authors suggest that a scalable prediction score for suicide in individuals with severe mental illness is feasible.
Summary
Using data on which factors tend to predominate in people with schizophrenia and bipolar disorder who end up dying by suicide, statistical methods are used to produce a tool, which you can use in a clinical situation to produce an estimated probability of dying by suicide. The paper reports the development and validation of one such clinical prediction tool, but says very little directly about how it might work in practice, e.g.
- How accurate would it be in a real-life mental health team?
- What might the adverse effects of giving clinicians such a tool to use, be?
- What are the appropriate cut-offs one should use?
The authors suggest that the tool might be used to identify low risk individuals who might be safely discharged, by providing a transparent quantitative summary of risk, with thresholds that might be changed from service to service.
Interventions to reduce suicide risk need to be prioritised according to need and risks, and this tool offers an approach to doing so. By providing the user with a probability score, it allows the application of different cut-offs, and balances between false positive and false negative predictions, based on the setting.
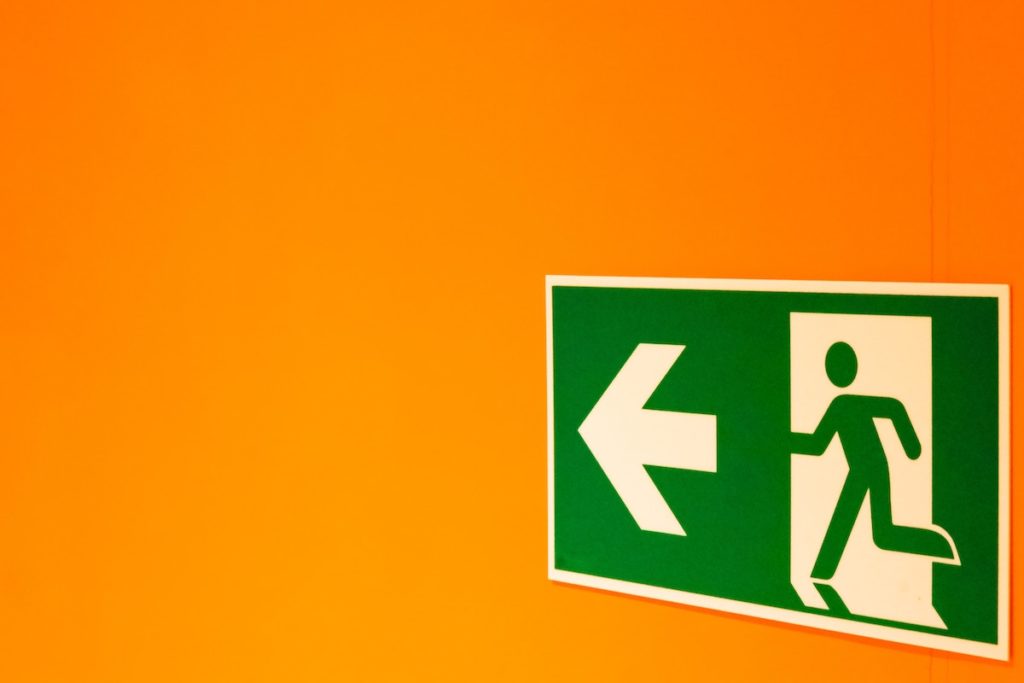
Could the OxMIS tool be used to identify low risk individuals who might be safely discharged, by providing a transparent quantitative summary of risk, with thresholds that might be changed from service to service?
Risk assessment in clinical practice: a summary |
1. Risk assessment (for suicide, physical violence, re-offending) is a core part of clinical practice in mental health, but lacks robust evidence. |
2. There are many tools, but little information on how tools are derived, including decisions made to include/exclude items, and performance. |
3. Absolutely no randomised evidence, limiting knowledge on real impact. |
4. Tools which are derived based on empirical data need performance assessed in external samples similar to the population in which the tool was developed. |
5. Tools should be developed transparently according to accepted rules, e.g. for number of outcomes, and in a way which allows for other investigators to replicate the work. |
6. It should be clear how variables included in the tool have been selected and usually should incorporate weights. |
7. It should be clear how the timeframe for the tool development study was selected. |
8. Measures need to be reported of how well the tool is calibrated (correctly estimates probability of the outcome in different groups within the population) and discriminates (distinguishes higher risk people from lower risk people). |
9. Tools should be easy to use and integrate into clinical practice. |
Conflicts of interest
I have no conflicts of interest to declare in relation to this blog.
Links
Primary paper
Fazel S, Wolf A, Larsson H, Mallett S, Fanshawe TR. (2019) The prediction of suicide in severe mental illness: development and validation of a clinical prediction rule (OxMIS). Translational Psychiatry https://doi.org/10.1038/s41398-019-0428-3
Other references
Jenkins P. (2014) Our risk averse mental health system is wasting money and harming recovery. The Independent Online; 2014.
Fazel S, Wolf A. (2018) Selecting a risk assessment tool to use in practice: a 10-point guide. Evidence-based mental health 2018;21(2):41-43.
Szmukler G, Rose N. Risk assessment in mental health care: Values and costs. Behav Sci Law 2013;31(1):125-140.
Photo credits
- Photo by Thomas Drouault on Unsplash
- Photo by NordWood Themes on Unsplash
- Photo by DDP on Unsplash
Haven’t suicide risk assessment tools been Recommended to not be used by NICE? And also, how clinically useful are the main predictors- gender, inpatient status, prep self harm are all known risk factors but don’t give much predictive power when sitting with an individual. No tool should ever replace a proper bespoke risk assessment with an individual.