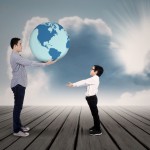
Uncovering the genetic component to common disease has been challenging. Whilst we now have more reliable heritability estimates (the proportion of susceptibility to disease which is accounted for by genetics), we are far from finding all genetic variants contributing to heritability. That is to say that if a disease is 80% heritable, we may have only uncovered genetic variants that account for 20% of this heritability.
The current methodology used is common variant association studies, also known as Genome-Wide Association Studies (GWAS). The principle behind GWAS is to use case-control analysis of common variants (defined as having a frequency of greater than 0.5% in the disease population) to signal regions of the human genome that are associated with disease. However, coverage using this methodology is limited and, in a recent paper, Zuk et al (2014) propose that using rare variant association studies (RVAS) rather than GWAS might be the key to uncovering the “missing heritability”.
Methods
Unlike GWAS, the design of RVAS has not been settled so they use a mathematical model to work out some of the fundamental factors that will enable RVAS to be statistically powerful enough to yield reliable and translatable results. The five factors they propose to test are:
- The choice of variant
- The frequency threshold
- Sample size
- Other strategies that could be employed
- Whole genome analysis
They started by investigating the choice of variant. Using a Bayesian model, they sub-categorise variants, in this case single base pair mutations, into three classes; silent, missense and disruptive:
- Silent mutations, i.e. those that do not affect protein structure, are classed as neutral
- Disruptive mutations, i.e. those that do affect protein structure, are classed as null
- Missense mutations are divided between the two depending on their level of disruption on protein structure
Interestingly, to mathematically model these missense mutations, they use evolutionary studies which have determined that 25% of missense mutations are disruptive, 50% are weakly disruptive (and called neutral) and 25% are neutral for all Mendelian diseases (think pea experiments by Gregor Mendel in the 1850s). These are then divided into two types; neutral and null, neutral having no effect on protein functioning and null disrupting protein functioning.
They started their modeling by using disruptive alleles only, in cases versus controls, where it was discovered they needed a vast sample size in order to keep high statistical power for the association tests. Next they investigated adding in null missense mutations. It was calculated that these would have to be selected for by frequency (so the frequency threshold would be lower and more strict) and severity in order to maintain a high power and reduce the need for a large the sample size.
Next they look at altering sampling, i.e. using isolated populations or selecting for gene sets (identified in GWAS) already known to be involved in common disease to see if it is easier to identify rare variants without a large sample size and with a higher frequency threshold. However both of these methods are limited, by studying isolated populations you are restricting the number of variants that you will discover due the relative nature of inter-relatedness of the population, so you will still need to look at case-control unrelated individuals in a large population sample to reveal the total genetic contribution to disease. Similarly with gene sets you are restricted to looking at genes that have been pre-identified so you may miss out on finding new genes involved in the disease, that have not been previously implicated.
Lastly, from the whole genome analysis, they looked at using de Novo mutations (or new mutations) which would need to have a large effect in order to be identified by the model or to look at non-coding regions, although they are less well defined functionally and so results may be difficult to interpret in terms of looking at biological or pathway models for disease.
Results and summary
Essentially the model they propose uncovered a lot of disadvantages to using rare variant association studies as the sole method for revealing genetic variants contributing to heritability. Unfortunately, rare variant association studies seem unlikely to become the gold standard in research in the next decade.
The most interesting finding was the sample size calculations. This is something that plagues GWAS in mental health too, for example, the latest GWAS in schizophrenia was conducted on 20,000 cases and 20,000 controls (approximately). This is still lagging behind other projects, for example height in over 100,000 samples, which only accounts for approximately 65% of heritability.
It seems that there is definitely something missing in the genetic heritability puzzle. It may be intronic (in non-coding regions of DNA), it may be epigenetic (selective methylation of DNA to turn genes “on” and “off” which changes across an individuals’ life time) or, in the case of psychiatric research, it may be endophenotypic (categorising individuals on the basis of abnormal biological responses rather than symptoms e.g. EEG waves).
In the meantime, however, this article highlights the need for more individuals to be involved in this kind of research, to give us the power we need for robust genetic heritability findings whilst we are methodologically limited to using these association studies to find the “missing heritability”.

Unfortunately, rare variant association studies seem unlikely to become the gold standard in research in the next decade
Links
Or Zuk, Stephen F. Schaffner, Kaitlin Samocha, Ron Do, Eliana Hechter, Sekar Kathiresan, Mark J. Daly, Benjamin M. Neale, Shamil R. Sunyaev, and Eric S. Lander. Searching for missing heritability: Designing rare variant association studies. PNAS 2014 ; published ahead of print January 17, 2014, doi:10.1073/pnas.1322563111
For a more comprehensive review of genetic association studies see: Lewis, C.M. & Knight, J. (2012) Introduction to gene association studies. Cold Spring Harb Protoc; doi: 10.1101/pdb.top068163).
Missing heritability: the final piece?: Uncovering the genetic component to common disease has been challengin… http://t.co/zmoBxLsHCd
Mental Elf: Missing heritability: the final piece? http://t.co/VaKRIkRvaf
‘Missing heritability’ in genetic research into psychiatric ‘disorders.’ It couldn’t be environmental factors…..??? http://t.co/yADLIqP2Ip
Alex Jarram highlights a new study which discusses the pros and cons of rare variant association studies http://t.co/R2qgVXTRVb
“We are far from finding all of the genetic variants that contribute to mental health heritability” http://t.co/R2qgVXTRVb
Why it is so impossibly complicated to figure out the genetics of mental disorders http://t.co/rEZ4IX8PdY @Mental_Elf
Missing heritability : enigma? http://t.co/g1u5HSUiJH
In case you missed it earlier: Missing heritability – the final piece? http://t.co/R2qgVXTRVb