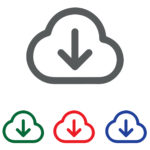
The title is deliberately provocative. Of course trials are crucial for establishing the efficacy of computerised therapies and apps for mental health. However, if we rely on evidence from trials alone, our idea of helpful digital therapies may be very out of step with what people are actually using ‘in the real world’.
For one thing, we may be unaware of the effects of publication bias (Torus et al., 2020). For another, online preferences change rapidly and research may lag behind. And, perhaps most critically, user engagement in digital tools in trials differs from engagement ‘in the wild’, outside of trial conditions. A handful of papers report digital mental health tool use outside of any formal trial or research setting (That is, not including any pre- or post-intervention assessments outside of the intervention itself, and not including any recruitment for trial purposes, nor including any implication that by using this program the user will be helping researchers or others with mental health needs.). Among these, uptake (reach or number of installs) varies enormously, but retention is routinely lower than for the same tools in trials (Baumel, Edan et al., 2019; Cohen & Torus, 2019; Fleming et al., 2018; Sanatkar et al., 2019).
There were over 90 million installs of mental health apps on Android devices by the end of 2018 (Baumel, Muench et al., 2019). Learning from such data is a massive opportunity. For example, assessing which types of apps people download and how much they use them can illuminate helpful directions for development. These might prevent clinicians and researchers putting large efforts into approaches that are seldom used.
This paper (Baumel, Munech et al., 2019) is the first to systemically examine usage patterns of self-help mental health apps using independently gathered internet traffic data. It aims to report features of unguided mental health apps associated with high reach and high retention.
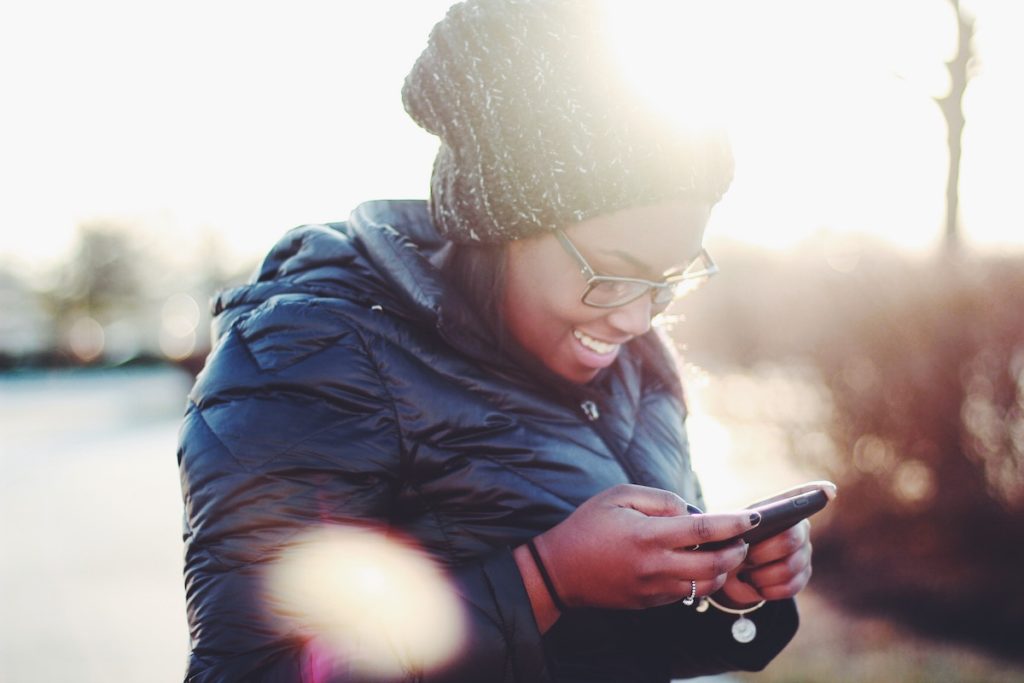
User engagement in digital tools in trials differs from engagement ‘in the wild’, outside of trial conditions.
Methods
The authors systematically searched for unguided apps for anxiety, depression or mental health (including mindfulness or happiness) available on the Google Play Store in November 2018. They included free apps (including those with optional in-app purchases) with over 10,000 installs that incorporated recognised techniques for self-management of mental health problems, symptom management or enhancing mental health.
Next (and here is the real coup), the authors obtained information on user traffic from SimilarWeb’s pro panel. SimilarWeb uses sources of anonymised data from consenting app users to provide aggregated, non-personal data on user engagement with websites and apps globally. The authors report that data gathering procedures comply with data privacy requirements and the data was consistent with other sources (e.g. Google Play Store data).
Results
The authors identified 386 unique mental health apps with over 10,000 installs. They excluded 87 apps based on description and a further 206 that did not incorporate evidence based techniques (79), did not have a relevant focus (62), had no data on SimilarWeb (23), or did not meet other inclusion criteria.
The remaining 93 apps comprised 59 primarily for a mental health problem, 8 for happiness, and 26 for emotional wellbeing. Nearly all contained components of cognitive behavioural therapy. Included therapeutic techniques were mindfulness /meditation (used in 43% of apps), mood trackers (54%), psychoeducation (41%), breathing exercises (29%), and peer support (used in 10% of apps).
Key points that may most interest developers, funders and advisors include:
- Install numbers varied widely. Some apps were installed over 10 million times, others little over 10,000 times. Overall, meditation and mindfulness apps where installed most frequently.
- A median of 4% of those who had installed an app within the previous 30 days opened it on any given day, with a median daily usage of 13 minutes. There was notable variation in median usage time. Apps incorporating peer support as a primary technique (just 2 apps) were used for a median of 35 minutes per day, mindfulness meditation apps for 21 minutes, and others for less than 10 minutes per day.
- Fifty-nine apps included data on 30-day retention. At day 15, a median of 3.9% of users opened the app. At day 30, the median figure was 3.3%.
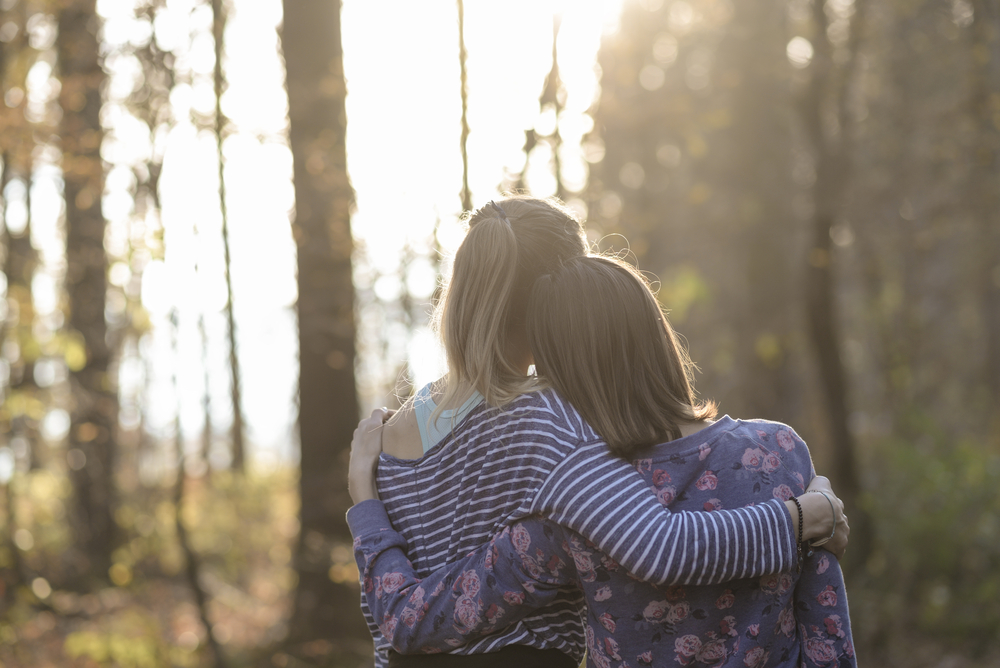
Some apps were installed over 10 million times. Apps incorporating peer support or mindfulness/meditation had the highest retention.
Conclusions
The authors demonstrate that mental health apps have wide appeal, but only a small proportion of users use the apps for long. Retention varies widely by app type, with apps incorporating peer support as a primary technique showing the highest engagement and mindfulness/meditation apps showing both high installs and moderately high engagement. These findings highlight promising directions for development.
The authors highlight that low retention rates may reflect poor engagement, but there may also be alternative explanations, e.g., an app’s purpose may be fulfilled quickly (e.g. for a breathing technique app). Importantly, where apps have millions of installs, even low retention can mean many people may be benefiting.
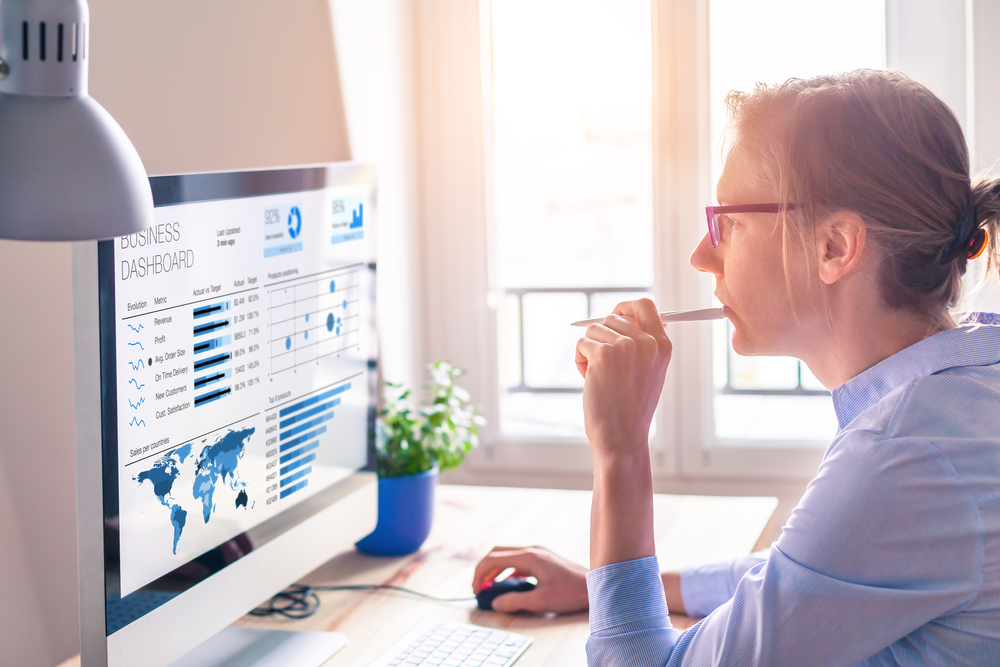
We should learn from apps with high installs and retention and make use of the massive data available from internet analytics.
Strengths and limitations
The strength of this paper is that is makes use of internet traffic data. Surprisingly, this is novel in digital mental health research. There are also important limitations, some reflecting the limitations of the data accessed. Is daily use of specific apps the most useful metric? It was the primary one available from the data source, but users might access multiple apps to create a suite of support, a possibility not identifiable in this data set. Users might install apps that are a poor fit for them, thus rapid drop out and installation of alternatives may be positive. Daily use might be less important clinically than metrics that were not available, for example weekly use. Further, there was no data about users’ needs or characteristics. Data were drawn from Android users who had allowed anonymised data tracking accessed by SimilarWeb, so they may differ from other users in important ways.
Other limitations reflect questions that the authors did not address within this study. For example, the paper did not include detailed analyses of features associated with high install rates. This is an important question for future research. App features could also be analysed in other ways. For example, were apps aimed at desirable states (mindfulness or wellbeing) more engaging than those aimed at reducing symptoms?
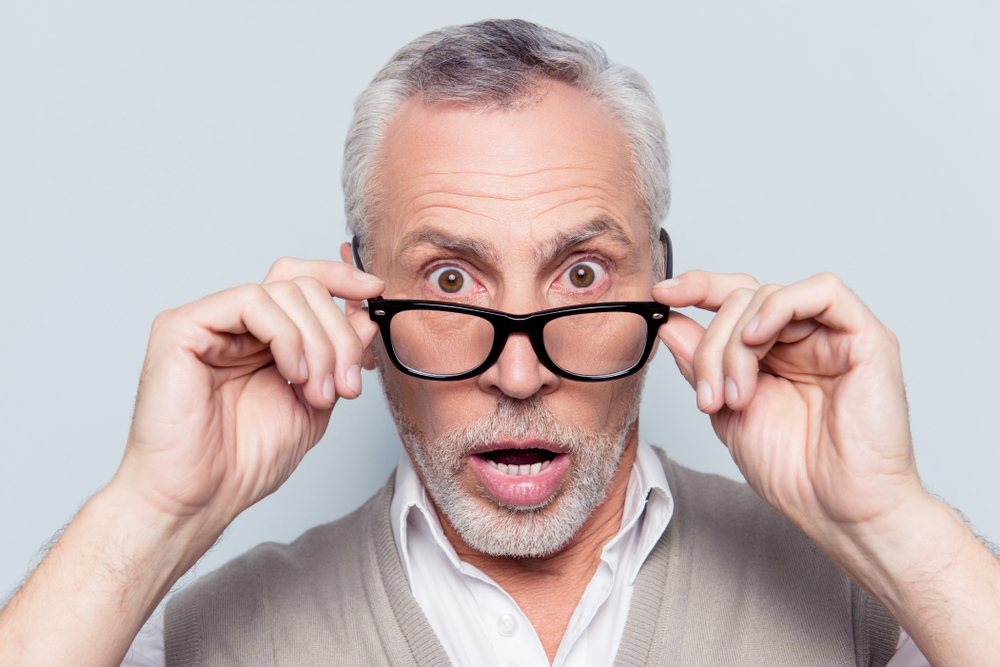
The strength of this paper is that is makes use of routinely collected internet traffic data, which surprisingly is a novel approach in digital mental health research.
Implications for practice
Practitioners and those involved in digital mental health should note that people are interested in installing mental health apps. The variation in install and engagement rates among different types of apps points to the importance of recognising differences between apps rather than assuming all operate similarly.
To me the most important finding is that we should make use of internet traffic data. I oversaw clinical aspects of the first three years of the New Zealand rollout of an unguided computerised CBT program for adolescents (SPARX) (Merry et al., 2012). It was striking how much public use of SPARX differed from that in trials (Burscheidt 2018, Merry et al., 2012, Fleming et al., 2012) (people completed it more quickly, dropout was higher and many users were outside the recommended age and symptom groups). At the time there were almost no published data with which to compare our findings, we now see that such patterns may be routine.
The research-implementation gap is not unique to digital therapies, however with digital interventions we have extraordinary potential to explore it. Routinely gathered data adds quite different insights from RCTs. If I was funding digital health tools, I would want to consider these data alongside that from controlled research. Otherwise we risk investment in tools that may differ vastly from those that people actually use.
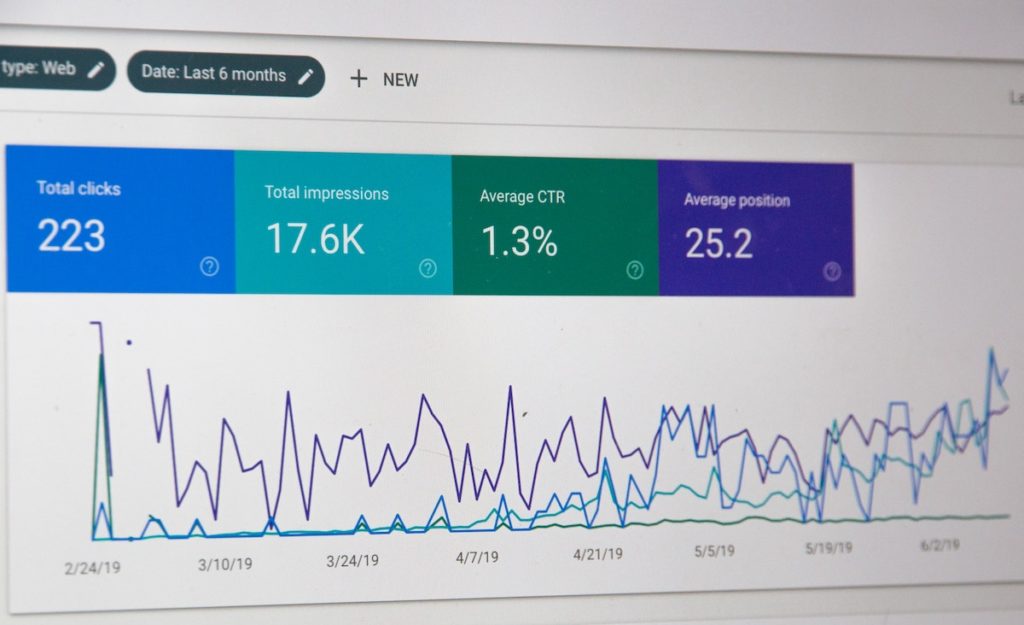
Alongside more traditional research, we should use internet analytics to understand how to maximise the potential of mental health apps.
Conflicts of interest
Terry Fleming is a co-developer of SPARX cCBT for adolescent depression. The IP for SPARX is held by Uniservices at The University of Auckland. The developers of SPARX can benefit financially from licensing or sales of the tool outside of New Zealand.
Links
Primary paper
Baumel A, Muench F, Edan S. et al (2019) Objective User Engagement with Mental Health Apps: Systematic Search and Panel-Based Usage Analysis. J Med Internet Res; 21(9): e14567 https://www.jmir.org/2019/9/e14567/
Other references
Baumel A, Edan S, Kane, J. (2019) Is there a trial bias impacting user engagement with unguided e-mental health interventions? A systematic comparison of published reports and real-world usage of the same programs, Translational Behavioral Medicine, 9 (6) 1020–1033 https://academic.oup.com/tbm/article/9/6/1020/5613435
Burscheidt L. Can gamified cCBT prevent depression in secondary school students? The Mental Elf, 10 Jan 2018. https://www.nationalelfservice.net/treatment/digital-health/can-gamified-ccbt-prevent-depression-in-secondary-school-students/
Cohen J, Torous J. (2019) The Potential of Object-Relations Theory for Improving Engagement With Health Apps. JAMA. 2019;322(22):2169–2170 https://jamanetwork.com/journals/jama/fullarticle/2755742
Fleming T, Bavin L, Lucassen M. et al (2018) Beyond the trial: systematic review of real-world uptake and engagement with digital self-help interventions for depression, low mood, or anxiety. J Med Internet Res 2018 Jun 06;20(6):e199 https://www.jmir.org/2018/6/e199/
Fleming T, Dixon R, Frampton C. et al (2012) A pragmatic randomized controlled trial of computerized CBT (SPARX) for symptoms of depression among adolescents excluded from mainstream education. Behav Cogn Psychother 40 5) 529-41 https://www.ncbi.nlm.nih.gov/pubmed/22137185
Merry S, Stasiak K, Shepherd M. et al (2012) The effectiveness of SPARX, a computerised self help intervention for adolescents seeking help for depression: randomised controlled non-inferiority trial. BMJ 18(344):e2598-e2516 https://www.bmj.com/content/344/bmj.e2598
Sanatkar S, Baldwin P, Huckvale K. et al (2019) Using Cluster Analysis to Explore Engagement and e-Attainment as Emergent Behavior in Electronic Mental Health. JMIR21 (11):e14728 https://www.jmir.org/2019/11/e14728
Torous J, Lipschitz J, Ng M. et al (2020) Dropout rates in clinical trials of smartphone apps for depressive symptoms: A systematic review and meta-analysis. Journal of Affective Disorders;263:413-9 https://www.sciencedirect.com/science/article/pii/S0165032719326060
Photo credits
- Photo by Meghan Schiereck on Unsplash
- Photo by Webaroo.com.au on Unsplash
[…] Here is my recent blog for the amazing Mental Elf on Amit Baumels new paper about what internet traffic data shows for mental health apps. In super-short: people behave differently in trials than they do once an app is ‘in the wild’ and there is wide variation in how much different mental health apps are installed and how much people keep using them. This has major implications for improving mental health. Hence my key point in this blog is that we should be using internet traffic data, alongside formal research data, to keep improving the sector. https://www.nationalelfservice.net/mental-health/digital-mental-health-tool-ignore-trial-read-analyt… […]
Really interesting paper and blog that includes some striking stats about use (good to know I’m not the only one who downloads mental well-being apps and hardly uses them – with the exception of a very simple breath app that has been supportive in stressful times!) For me this raises some questions about the pros & cons for users of impersonal provision by way of apps, and personalised digital provision eg online therapy. For example, might easy access apps be a helpful gateway to something initially more daunting such as working with a counsellor or therapist? And are there therapeutic advantages of impersonal apps over personalised support? Question for the author if you have a view on this – what outstanding literature would you recommend a newcomer to digital mental health field should read to start becoming well informed?