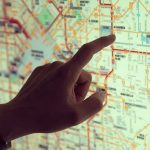
Depression is common (Otte et al., 2016; World Health Organisation), accounting for the largest proportion of disability-adjusted life years (DALYs) among mental health diagnoses (GBD 2019 Mental Disorders Collaborators, 2022).
There are multiple ways to define and measure depression, all of which rely on the assessment of symptoms. For example, according to the Diagnostic and Statistical Manual of Mental Disorders (DSM-5; American Psychiatric Association, 2013), an individual suffering with depression will show at least five of nine pre-defined symptoms within a two-week period, one of which must be low mood or anhedonia (the lack of interest in or enjoyment of activities).
However, people with depression vary greatly in the number and combination of symptoms they experience. In fact, numerous combinations of symptoms satisfy the DSM-5 criteria for depression, leading to huge variability in clinical profiles. For instance, hundreds of unique patterns of symptoms were identified in one large sample of adults with depression (Fried & Nesse, 2015). Research focusing on individual symptoms has reinforced this conclusion, and further suggests that specific symptoms are differentially associated with psychosocial impairment (Fried & Nesse, 2014). Importantly, symptoms may also exist in dynamic relationships (Borsboom, 2017): that is, individual symptoms can affect one another. For example, insomnia may lower concentration levels which in turn may cause feelings of low self-worth. Importantly, two individuals with the same recognised overall severity of depression and/or similar symptom profiles could show very different relationships between symptoms. However, research has hitherto devoted little time to exploring individual differences in these ‘symptom dynamics’.
This study by Omid V. Ebrahimi and colleagues (2024) examined depression symptom dynamics by combining ecological momentary assessment (EMA) and network analysis. In EMA, participants’ mood and behaviour are repeatedly sampled in their everyday environment, in real time throughout the day. In network analysis (an item-level statistical framework for psychological variables) each symptom is represented by a node, and relationships between symptoms are represented as edges between nodes, allowing symptom dynamics to be quantified over time.
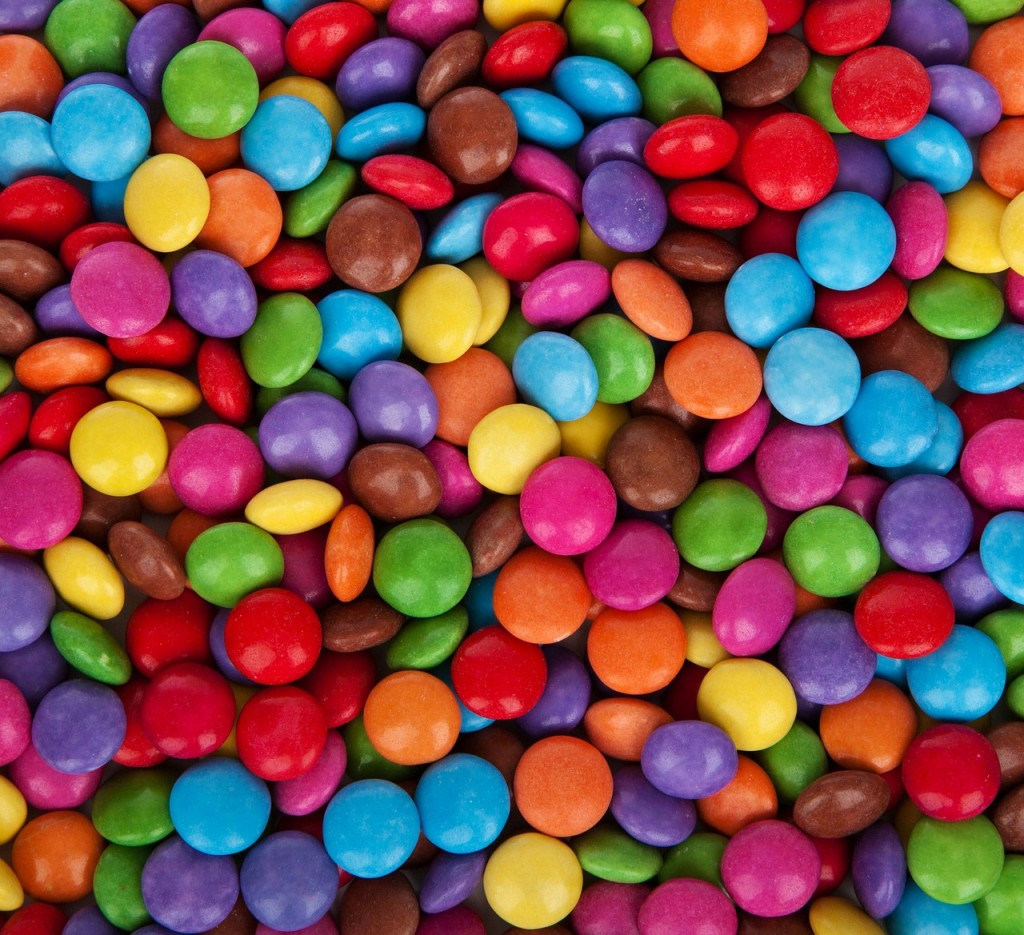
People with depression vary substantially in the number and combination of symptoms they experience leading to huge variability in clinical profiles.
Methods
Ebrahimi and colleagues used data from the ZELF-i randomised controlled trial (Bastiaansen et al., 2018), which investigated the effects of self-monitoring depression using EMA. Eligible participants (n=74) were aged between 18-65 years and had been diagnosed with depression by a clinician. Depression severity was assessed with the self-reported Inventory of Depressive Symptomatology (IDS-SR). Participants were prompted to record their mood five times per day over 28 days, during 3-hour time windows. EMA mood items were matched to depression symptoms and were scored on a visual analogue scale (ranging 1-100).
To analyse the data, dynamic network analysis was used to estimate individual-specific networks using a technique called the “graphical vector autoregressive model” (GVAR). This model yields two networks for each person:
- The “temporal” network, which represents the impact of each symptom on other symptoms at a later time point (in this case, three hours later).
- The “contemporaneous” network, which represents associations between symptoms after accounting for temporal relationships, occurring within the same 3-hour time window.
Once these networks had been estimated for each person, the authors compared networks from different individuals with identical overall severity scores to assess the prevalence of differences in network dynamics. To do this, they used a statistical technique called the “individual network invariance test” (INIT). This test involves either setting the edges in networks to be equal across individuals or allowing them to vary, and then assessing the evidence for each model. Additionally, extensive simulations were performed to investigate possible biases in network comparisons due to sample size, missing data, and response rates.
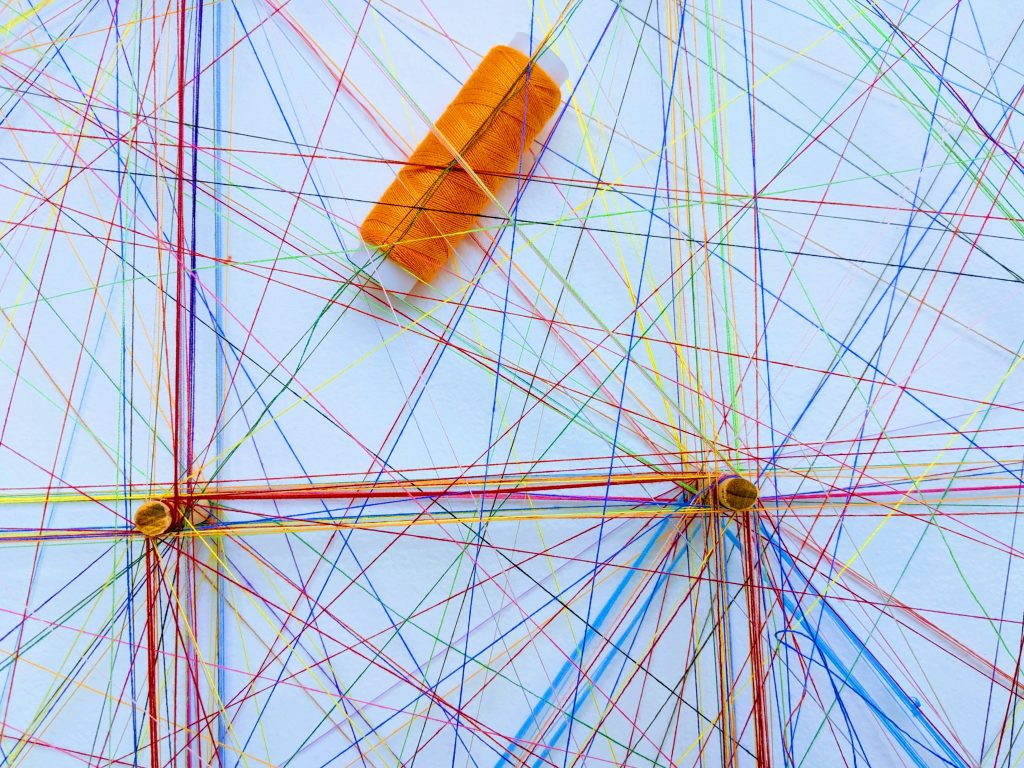
This study used network analysis and ecological momentary assessment to explore relationships between symptoms of depression over time, comparing participants with the same overall depression severity to one another.
Results
A total of 74 participants between 18 and 64 years old were included in the study (on average around 34 years old), and just over half of the sample (56.16%) identified as female. Overall, the most frequently reported level of depression severity was ‘severe’ (i.e., participants most frequently scored more than 31 out of a possible 84 on the IDS-SR). Twenty-three different depression severity levels were identified. Each of these levels included at least two participants, with a maximum of six participants in each level.
The headline result of the paper was that 63% of participants that matched on overall symptom severity showed different symptom networks, as assessed by INIT. For example, two participants had a depression severity score of 31 (out of a possible 84), and were matched on age (23-24), gender (female) and educational attainment (had at least completed a high-school education). The temporal networks for these two participants showed that while in one participant the symptom of lethargy preceded the symptom of anhedonia, in the second participant anhedonia preceded lethargy. Similarly, the symptom of restlessness preceded depressed mood in the first participant, while the opposite was the case for the second participant.
Interestingly, two core symptoms of depression, anhedonia and depressed mood, affected each other in a mutually reinforcing cycle (a ‘vicious cycle’), with each symptom increasing the level of the other over time. However, this was only true in some of the participants with the same overall depression severity, and was absent in other participants. This exemplifies the evidence that even when participants were matched on overall severity, there were differences in the underlying relationships between symptoms. In other words, although participants may have been similar in demographic characteristics (like age, gender and education), and depression severity (severe depression), focusing on individual symptoms of depression, and particularly the associations between them over time, revealed potentially important differences in symptom dynamics.
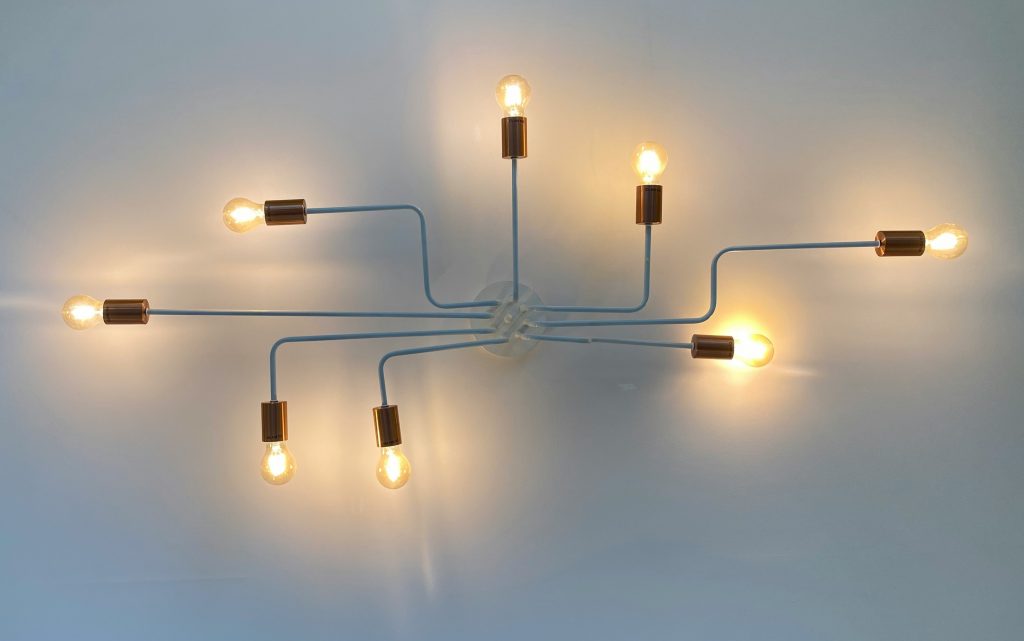
63% of participants who had the same overall depression severity, showed differing symptom networks (relationships between individual symptoms).
Conclusions
This paper provides clear evidence that the relationships between depressive symptoms vary between individuals with depression who are matched on overall depression severity. This provides unique insight into an important source of clinical heterogeneity in depression. The authors suggest that taking into consideration the relationship between individual symptoms over time might be an important way of characterising depression in individuals, and may be key to the development and tailoring of personalised interventions.
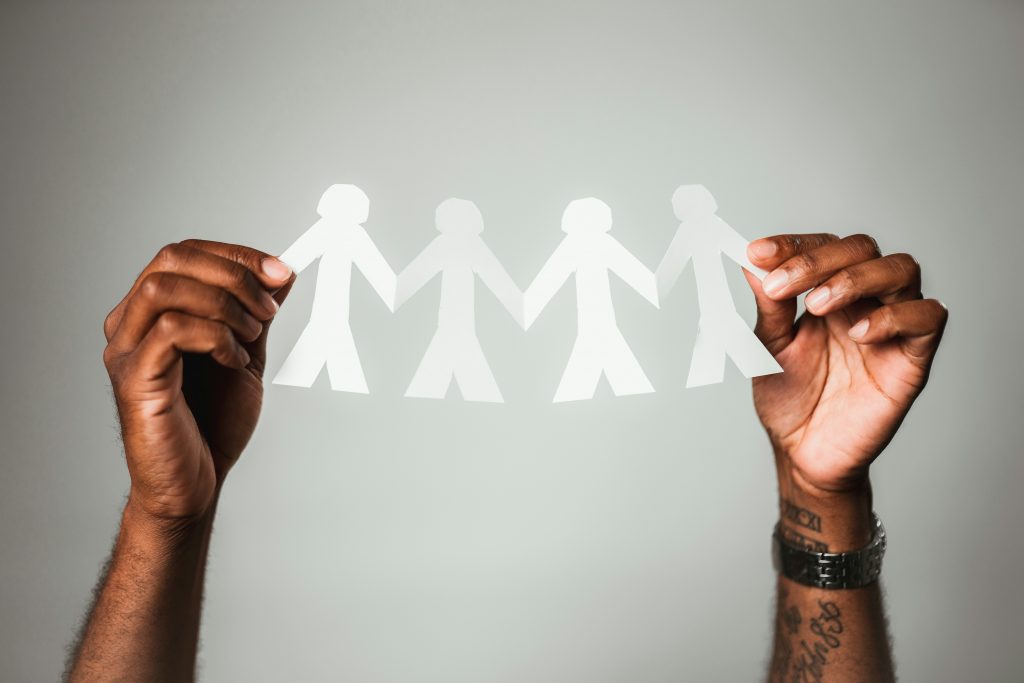
This study provides evidence that there are substantial individual differences in how individual symptoms of depression interact with each other over time.
Strengths and limitations
This paper was descriptive in design, providing a proof of principle of the existence of individual differences in symptom dynamics between people with depression. The dataset for within-person analyses is substantial, complemented by a thorough and rigorous investigation of symptom dynamics, sensitivity analyses with simulations, and open access to all code and materials. As the authors note:
The proportion of individual differences in symptom dynamics is likely to have been underestimated, given the method’s conservativeness
… meaning the actual differences are likely much larger than those presented in this paper. The sample size is moderate for between-person analyses, and only 23 (out of a possible 84) depression severity levels were identified.
As in all network analyses, the precise pattern of results will depend on the choice of nodes. Importantly, some key symptoms of depression were unavailable in this dataset (e.g., concentration and sleep problems, feelings of worthlessness, and suicidal thoughts). In particular, concentration problems are known to contribute substantially to functional impairment (Fried & Nesse, 2014), and sleep problems are associated with antidepressant treatment (Boschloo et al., 2019). It would be important to include these symptoms in future investigations to characterise depression dynamics more completely.
Participants were matched on overall symptom severity, assessed by total score on the IDS-SR. However, symptoms of depression are heterogeneous, and summary scores often neglect this important source of variability. Matching participants on their symptom profiles (either exactly or with similar symptom combinations) is a potential alternative approach that would provide a more convincing demonstration of the value of network dynamics over and above existing measures. However, this would require much larger sample sizes than currently available in most EMA studies.
The authors conclude that there are substantial individual differences in how depression symptoms interact with each other over time. In other words, by focusing on individual symptoms, the study finds great variability in associations between symptoms over time across individuals, revealing a potentially important source of heterogeneity. Disentangling this heterogeneity might help to more accurately describe an individual’s experience of depression. However, it remains to be seen whether symptom dynamics are important in relation to predicting either one’s evolution of depression (e.g., remitting, relapsing or chronic) or response to treatment.
Implications for practice
This study described a new way of characterising fluctuations in individual symptoms of depression, and applied a novel statistical procedure to rich, time-intensive data. This symptom-level approach is still in its early stages, which precludes drawing clear clinical implications from the authors’ findings.
However, the study does open up potentially promising avenues for future research, which could improve the precision of psychological assessment and subsequent selection of treatment. For instance, monitoring the development of symptoms of depression, and the extent to which symptoms of depression affect each other, could help identify people who would benefit from rapid, time-sensitive interventions, perhaps targeted at particular symptoms. This study also stresses the importance of recognising the heterogeneity between individual experiences of depression and the potential effect of this on patient responses to treatment.
In summary, characterising the relationships between symptoms has the potential to help us further our understanding of important dynamics in the course of depression, and may help us better characterise how depression manifests in a given individual. Monitoring the temporal fluctuations of symptoms may provide useful information on maladaptive associations between symptoms, for both clinicians and individuals experiencing depression.
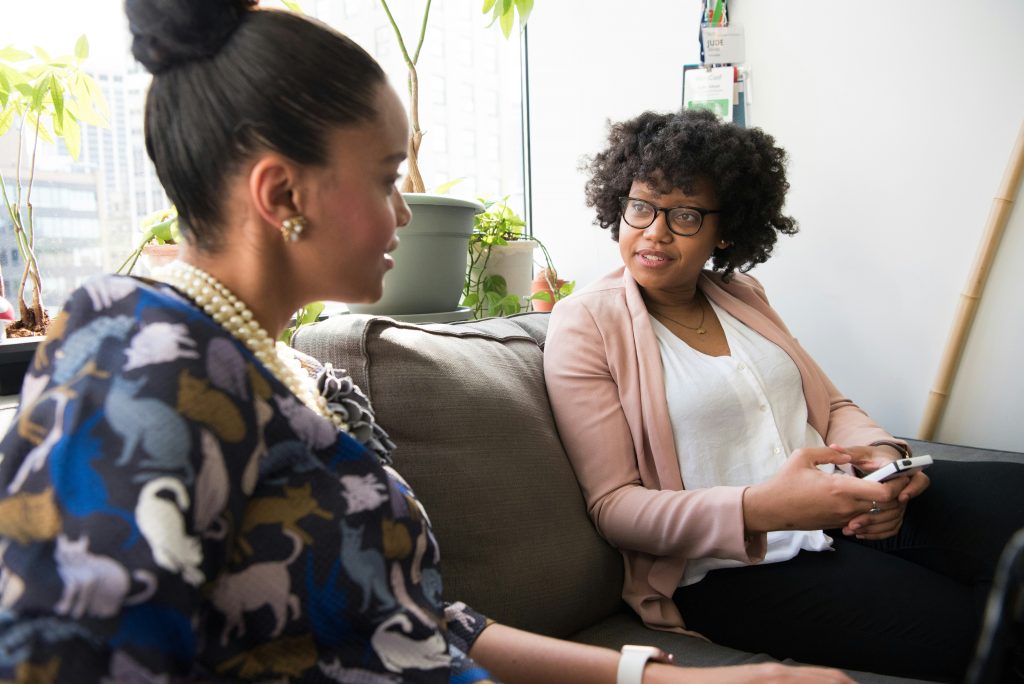
Understanding how symptoms interact over time may help patients and clinicians to better describe and monitor the episode of depression being experienced.
Statement of interests
Giulia Piazza and Jonathan Roiser have previously co-authored a network study with Sacha Epskamp, a co-author of the paper discussed in this blog.
Links
Primary paper
Ebrahimi, O. V., Borsboom, D., Hoekstra, R. H. A., Epskamp, S., Ostinelli, E. G., Bastiaansen, J. A., & Cipriani, A. (2024). Towards precision in the diagnostic profiling of patients: Leveraging symptom dynamics as a clinical characterisation dimension in the assessment of major depressive disorder. The British Journal of Psychiatry, 224(5), 157–163.
Other references
American Psychiatric Association. (2013). Diagnostic and Statistical Manual of Mental Disorders (5th ed.).
Bastiaansen, J. A., Meurs, M., Stelwagen, R., Wunderink, L., Schoevers, R. A., Wichers, M., & Oldehinkel, A. J. (2018). Self-monitoring and personalized feedback based on the experiencing sampling method as a tool to boost depression treatment: A protocol of a pragmatic randomized controlled trial (ZELF-i). BMC Psychiatry, 18(1), 276.
Borsboom, D. (2017). A network theory of mental disorders. World Psychiatry, 16(1), 5–13.
Boschloo, L., Bekhuis, E., Weitz, E. S., Reijnders, M., DeRubeis, R. J., Dimidjian, S., Dunner, D. L., Dunlop, B. W., Hegerl, U., Hollon, S. D., Jarrett, R. B., Kennedy, S. H., Miranda, J., Mohr, D. C., Simons, A. D., Parker, G., Petrak, F., Herpertz, S., Quilty, L. C., … Cuijpers, P. (2019). The symptom-specific efficacy of antidepressant medication vs. cognitive behavioral therapy in the treatment of depression: Results from an individual patient data meta-analysis. World Psychiatry, 18(2), 183–191.
Fried, E. I., & Nesse, R. M. (2014). The impact of individual depressive symptoms on impairment of psychosocial functioning. PloS One, 9(2), e90311.
Fried, E. I., & Nesse, R. M. (2015). Depression is not a consistent syndrome: An investigation of unique symptom patterns in the STAR*D study. Journal of Affective Disorders, 172, 96–102.
GBD 2019 Mental Disorders Collaborators. (2022). Global, regional, and national burden of 12 mental disorders in 204 countries and territories, 1990–2019: A systematic analysis for the Global Burden of Disease Study 2019. The Lancet Psychiatry, 9(2), 137–150.
Otte, C., Gold, S. M., Penninx, B. W., Pariante, C. M., Etkin, A., Fava, M., Mohr, D. C., & Schatzberg, A. F. (2016). Major depressive disorder. Nature Reviews Disease Primers, 2(1), Article 1.
World Health Organisation. Depressive disorder (depression). Retrieved 22 November 2023.
Photo credits
- Photo by José Martín Ramírez Carrasco on Unsplash
- Photo by Kelly Sikkema on Unsplash
- Photo by Omar Flores on Unsplash
- Photo by Fabio Bracht on Unsplash
- Photo by Andrew Moca on Unsplash
- Photo by Christina @ wocintechchat.com on Unsplash