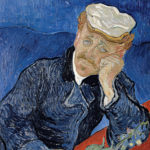
A group of authors led by teams at University of Edinburgh and Kings College London have posted a new genetic analysis of depression (genome-wide association study; GWAS) on the bioRxiv website (Howard et al, 2018), where biomedical research papers can be posted prior to peer-review and publication. Five years ago, analyses of previously unthinkable numbers of cases and controls (tens of thousands) showed no statistical associations between depression and any of the millions of locations in the genome where DNA sequence can vary. Now, hundreds of genes are implicated. The analyses are technically complex and the papers are dense and full of statistical terminology. I will try to make the methods and results a bit more accessible here.
The principle of GWAS is that “SNP chips,” which assay hundreds of thousands of variant loci, actually provide information about millions of loci: in each short DNA segment only a few common combinations of variant sequences actually occur, so that assaying a few loci in each segment allows “imputation” (mathematical prediction) of the rest (around 8 million). GWAS chips are cheaper (currently <$100) than full genome sequencing (>$1000), and require less time and computer power to analyse. Thus, very large cohorts are feasible.
This is fortunate, because “common variants” (at least 1% of chromosomes have the “different” sequence) have small effects on human traits and diseases, whereas more pathological variants die out or remain quite rare exactly because they are harmful and thus reduce survival or fertility. Depression is one of many “polygenic” traits: around 30-40% of the tendency to become depressed is genetic, and a significant part of that is the combined actions of many variants. Here, common SNPs explained, in total, around 9% of the total risk of depression. (Studies using much narrower definitions of severe depression, diagnosed by direct interviews, show that SNPs probably explain larger proportions of risk, but it is impossible to make these studies sufficiently large to obtain significant results for very many genes.)
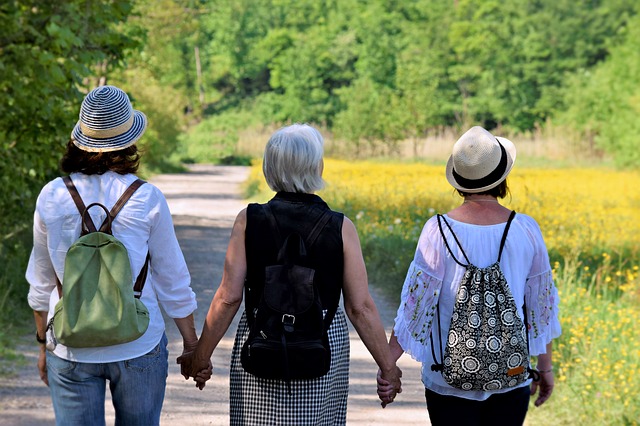
Around one-third of our tendency to become depressed is genetic.
Methods
These authors have combined the data from the three largest genetic analyses of depression: one from the 23andMe company (~307,000 people), one from the UK Biobank (361,000) and one from the Psychiatric Genomics Consortium (139,000), totalling 807,553 people including 236,363 with depression (after removing duplicate or related individuals). One might ask: how did the researchers interview so many people? They didn’t. Some of the PGC cohorts were interviewed, while others used information from electronic medical records. But 23andMe and UKB simply asked participants online if they had been treated for depression or similar problems. One can question the latter methods, but the authors found correlations of the statistical results across the genome were >0.85 between pairs of studies regardless of diagnostic method, i.e., they are picking up the same genetic “signal.”
(Some additional clinically-relevant information: whereas 23andMe asked their highly educated clientele questions like “Has a doctor ever given you a diagnosis of clinical depression?”, the UK Biobank study relied on a very general question about whether the individual had ever been treated for “depression, nerves, anxiety or tension”. They found that among people who answered yes to this question and for whom more detailed information was available, 64% had evidence of major depressive disorder, and most of the rest had some form of anxiety or depressive problem or a high neuroticism score. This question constituted the data variable that was available for the largest proportion of the entire UKB cohort. The investigators reasoned that, given that a broad definition of depression was inherent to their analysis because of the strategies of other studies such as 23andMe, they would achieve the greatest power with this very broad question. Further, both twin and previous GWAS analyses have shown a very high genetic correlation among non-bipolar depressive and anxiety disorders (with a significant but much lower correlation with bipolar disorders). I share their conclusion that this was a reasonable choice in this context; discovering the largest possible number of associations that predispose to depression during one’s lifetime. However, it will require other strategies to determine, e.g., whether predisposition to the most severe forms of clinical depression might be associated with a partially different set of genetic variants, and whether this “broad depression” strategy might over-estimate the correlation of common-variant factors among the broader set of depressive and anxiety problems).
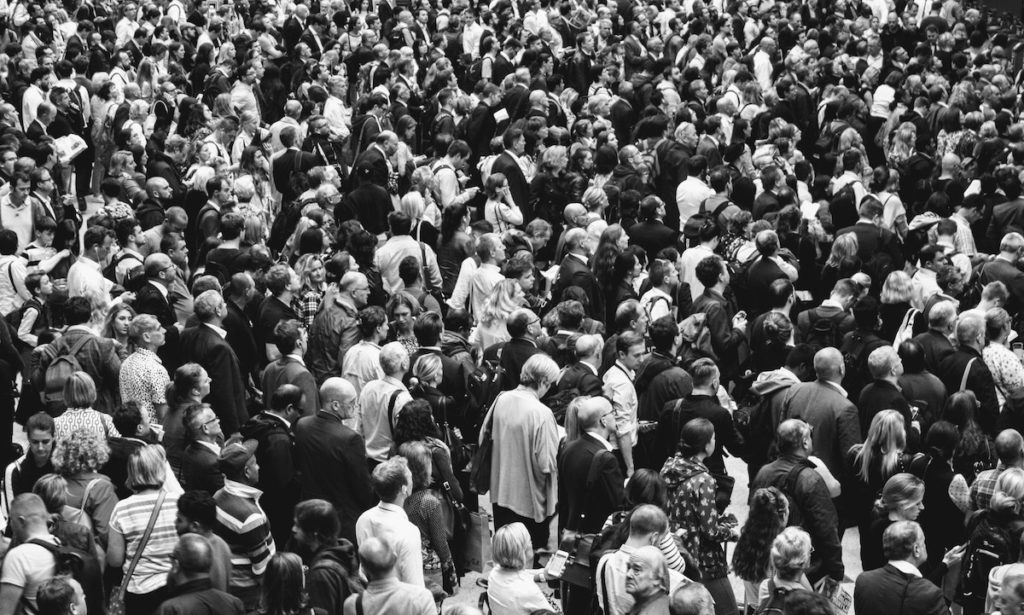
This study meta-analysed data on 807,553 individuals (246,363 cases and 561,190 controls) from the three largest genome-wide association studies of depression.
Results
The results of the three studies were combined via a statistical technique called meta-analysis. For each of the variable genetic locations, meta-analysis takes the statistical result from each study, weights it to reflect the sample size, and computes a combined statistic. There is a generally-accepted statistical threshold of significance for GWAS data (p<5 x 10-8) based on estimates of the number of “independent” tests across the genome (around 1 million). Here, 102 sequence variants (single-nucleotide polymorphisms; SNPs) were significant and statistically independent of each other, compared with 15 (23andMe), 17 (a smaller UKB analysis) and 44 (PGC including smaller parts of 23andMe and UKB).
Then, the significance of evidence for association in each gene was tested by combining results from nearby SNPs while accounting for the correlations among those SNPs. DNA variation in 269 genes significantly predicted depression. Each gene has only a small effect, so it takes many of them to produce a high risk of depression. An additional analysis then determined whether evidence for association was “enriched” (greater than chance) in gene “sets” which have specific biological functions (although it is well-known that these “annotations” are not complete). There were 14 partially-overlapping significant sets, mostly related to the structure and activity of synapses (the connections between neurons) or responses to biological stimuli.
Finally, an analysis examined whether the more significant results reflected the profiles of gene expression (activation) in specific tissues, and enrichments were seen for profiles associated with brain cells, and particularly the neocortex and the frontal cortical regions. That might be expected for a psychiatric disorder, but there was a notable absence of evidence for a role of immune dysfunction which is considered a possible factor in depression. Although the PGC analysis highlighted genes that were active both in neurons and in the immune system, the new analysis provides little support so far for the latter. Perhaps other analytic approaches will clarify this in the future.
Mental health professionals and patients may be particularly interested in the analysis of whether the implicated genes were relevant to the actions of known drugs, using an online database of relevant information. There were 560 interactions involving 57 of the “depression genes” and 514 drugs. Some of these are psychiatric drugs, like antipsychotics (which sometimes have, or augment, antidepressant effects), but others are either experimental drugs with novel targets (like the ErbB4 receptor of the Neuregulin 1 protein), or drugs that are currently considered to have no role in treating depression.
Given that no new antidepressant drug mechanisms have been discovered for decades, and that currently-available drugs are far from ideal for many patients, one of the major goals of depression genetics research is to identify new drug targets for antidepressant drug discovery. These new results suggest many new options. The authors note, however, that genes related to serotonin are notably absent, despite the current prominence of antidepressants with serotonergic effects. Thus, treatments do not always have to be linked to genetic etiological factors, but it is hoped that drugs targeting the latter could have more specific and consistent antidepressant effects.
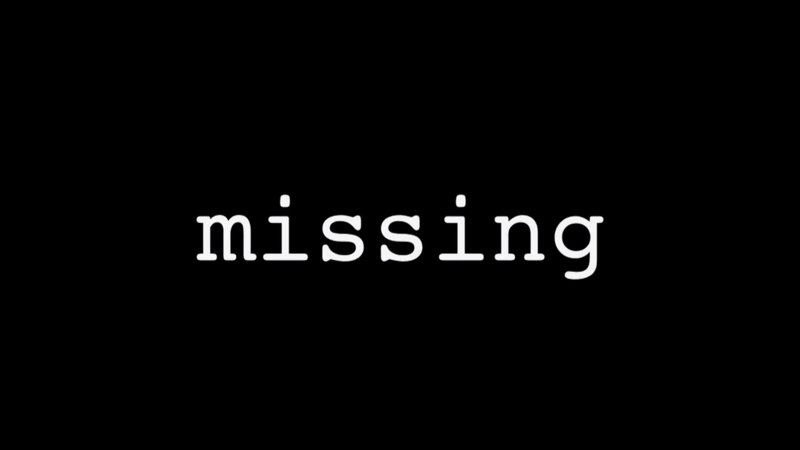
Genes related to serotonin are notably absent, despite the current prominence of antidepressants with serotonergic effects.
There are too many analyses in the paper than can be summarised here, including possible genetic relationships with other psychiatric disorders and with non-psychiatric diseases.
Take-home messages
- Depression is tractable to modern genetic research methods.
- Extraordinarily large cohort sizes have been needed, which has been feasible because of drastically reduced genotyping costs and more efficient (although abbreviated and less precise) phenotyping methods.
- Increased cohort sizes yield new association findings, a pattern that can be predicted to continue for the foreseeable future.
- There are now enough associated genes to begin to recognise patterns in the gene networks and cell types that underlie depression risk; within the limits of current knowledge about gene functions and the lack of available large-scale data about rarer genetic variation.
- The associated genes suggest new avenues for antidepressant drug development: new targets; known targets for which experimental drugs are already under study; and known drugs whose relevance to depression treatment has not been previously appreciated.
- Larger meta-analyses improve power to calculate genetic correlations between depression and other phenotypes, which further aids discovery of treatment strategies.
- Challenges remain about understanding the effects of including such a broad range of depression “cases” in these analyses, and for extending these studies to population groups other than people of predominantly European ancestry. But so far, the high correlations among datasets with very different phenotyping strategies, for both genome-wide results and top findings, suggest that the current strategy is yielding important new information about depression. One hopes that investigators will continue to be able to expand their datasets for depression and related problems, both broadly and narrowly defined, to make further progress.
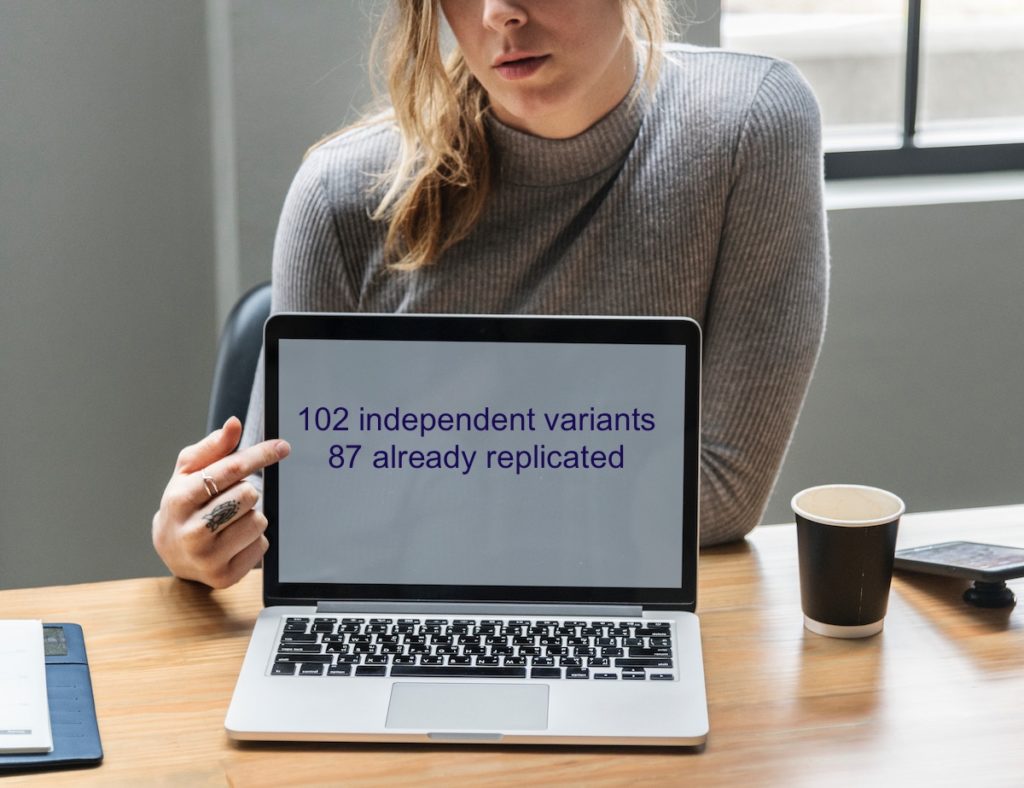
There are now enough associated genes to begin to recognise patterns in the gene networks and cell types that underlie depression risk.
The #GLADstudy
While you are here, please take a look at the Genetic Links to Anxiety and Depression (GLAD) Study which hopes to change the landscape of research into depression and anxiety in the UK. This initiative involves recruiting 40,000 participants who have experienced clinical depression or anxiety during their life into a recountable database of participants to enable future studies on these conditions, particularly with regard to treatment outcomes. Taking part involves signing-up online, providing consent, completing an enrolment questionnaire and providing a saliva sample through the post.
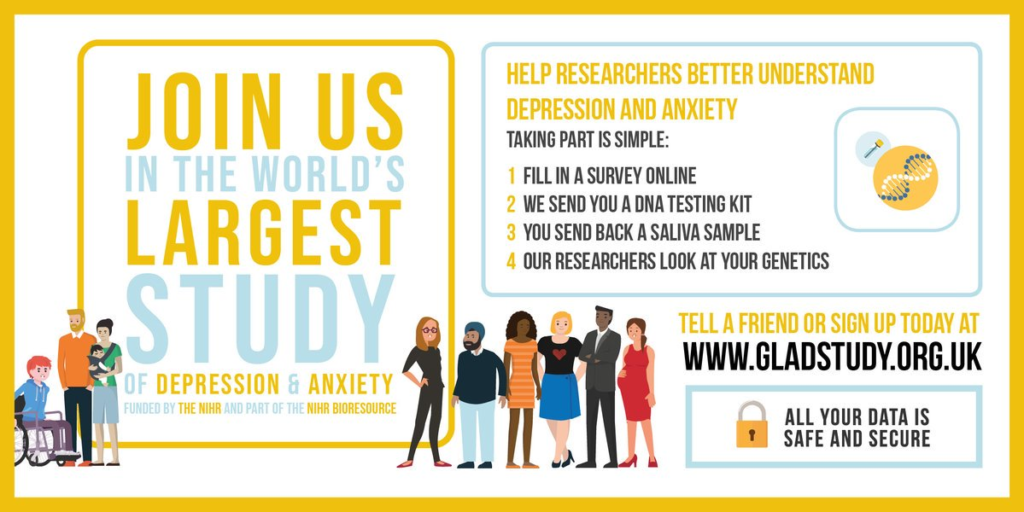
The GLAD study is recruiting now! Find out more at gladstudy.org.uk
Links
Primary paper
Howard DM. et al (2018) Genome-wide meta-analysis of depression in 807,553 individuals identifies 102 independent variants with replication in a further 1,507,153 individuals. bioRxiv 433367; doi: https://doi.org/10.1101/433367
Photo credits
- Photo by Rob Curran on Unsplash
- Photo by rawpixel on Unsplash
For the record, there was no actual replication of previous findings, nor did they perform an actual independent GWAS on the 23andMe replication dataset to compare significant variants. In short, to date there has not been a single independently replicated, significant genetic variant for depression. At this point, each new dataset is rolled into the previous as a meta-analysis, so actual independent replication isn’t even attempted for most GWAS studies of mental disorders.
Of the 44 genome-wide significant variants reported in a previous study by Wray et al. (2018), 38 were available across all cohorts in our paper. 38/38 had the same direction of effect in 23andMe. 38/38 had the same direction of effect in UK Biobank and 38/38 had the same direction of effect in the Meta-analysis.
Of the 102 variants identified in our meta-analysis, 87 were significant in the replication cohort (after multiple testing) and many were also genome wide significant in the 23andMe independent replication data set (see supplementary table 1). We were also able to show, by calculating genetic risk scores in an independent sample, that people with higher scores (calculated on the basis of the meta-analysis) were at higher risk of major depressive disorder.
There’s so much more to do, but the results are replicating across studies – an important issue usefully highlighted by the comment above. Its a very exciting time to be doing depression genetics research.
We cover some of the issues and future priorities in a new paper, published yesterday, which you can find here: https://authors.elsevier.com/a/1YqUA3BtfGtEh7