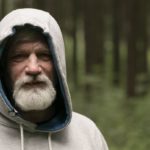
Around 50 million people worldwide are living with dementia (Alzheimer’s Disease International, 2015), the most common cause of which is Alzheimer’s disease (AD). The burden of this disease on an individual level is considerable; memory and functional decline are well-known core features but a significant number of people also experience depression, anxiety, agitation and psychosis, even if they did not have these symptoms before their diagnosis.
At a societal level, AD and other dementias are thought to cost the UK economy more than £25 billion, with two thirds of that cost borne by people with dementia and their families in the form of unpaid care or private social care (Alzheimer’s Society, 2014). There are no disease modifying therapies and the last licensed treatment for symptomatic relief was memantine, more than 15 years ago.
So, it’s easy to see why there is such a focus on trying to get a handle on disease mechanisms underlying AD and genetic studies have played an important role here. The genetics of Alzheimer’s disease is a pretty well established area and the gene most strongly associated is APOE, which we have known about since the early 90s. In the last decade there has been success in moving beyond APOE to the identification of other genes robustly associated with the disease (Harold et al. 2009; Lambert et al. 2013). These increasingly large studies, examining the whole genome, have been pivotal in shifting focus in AD towards immune and inflammatory pathways, and so steering drug target identification.
This latest genetic analysis of AD by Jansen et al. (2019) is the largest ever to be conducted. It aims to shed further light on biological pathways and other disease mechanisms implicated in AD. It takes the results of prior studies, combines them with new data (known as meta-analysis) and then uses smaller independent samples for replication. In total, data from over 450,000 people were used.
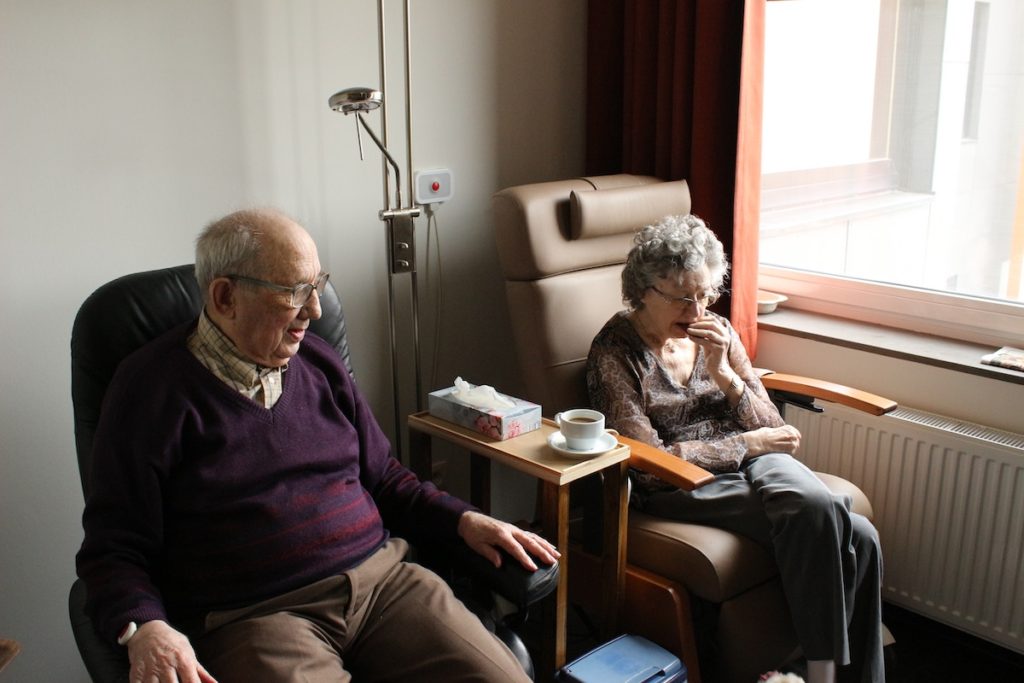
Alzheimer’s disease costs the UK over £25 billion per year, the majority of which is borne by people with dementia and their families.
Methods
The study design used is known as ‘case/control’ and the method used was a genome-wide association study (GWAS). Here, people are either assigned to ‘case’ status (AD) or ‘control’ status (no AD). Millions of genetic variants are profiled in each person and researchers then analyse whether the frequencies of each one are different in cases vs controls. If they are, it can be said that the variant in question is associated with disease.
Data from over 450,000 people were analysed in three phases. AD status was either derived from clinical diagnoses or by proxy, which in this case means an individual’s AD family history status (so if someone has a biological parent with a history of AD they are assigned ‘case’ status and if someone has no family history of AD they are assigned ‘control’ status).
Phase 1 was a meta-analysis of three different case/control studies. Phase 2 was an analysis of the proxy AD phenotype using UK Biobank data. Phase 3 was a meta-analysis of the findings from phase 1 and 2 and the top statistically significant hits were then tested for replication in an independent cohort.
Finally, a series of bioinformatic tools were used to help place the findings in functional context and examine whether AD is genetically related to a range of other heritable traits (both medical and non-medical: e.g. educational attainment and height).
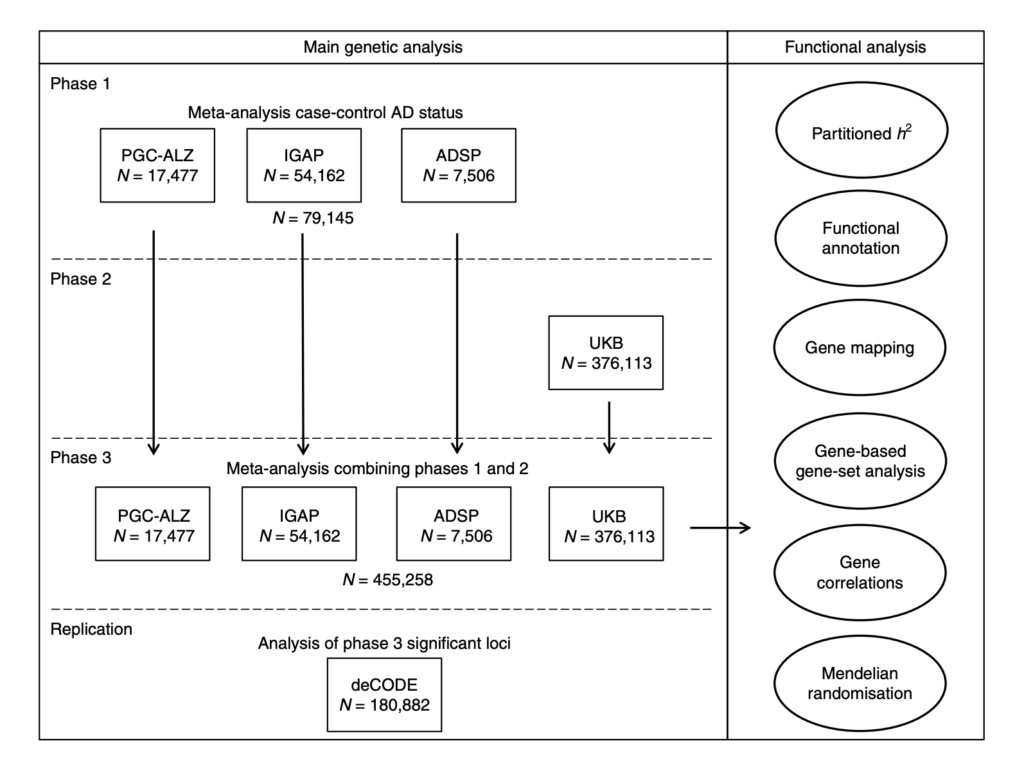
Fig. 1 Overview of analysis steps. The main genetic analysis encompasses the procedures to detect GWAS risk loci for AD. The functional analysis includes the in silico functional follow-up procedures with the aim to put the genetic findings in biological context. N= total of individuals within specified data set.
Results
The results from phase 1 are more or less a replication of previous large genetic studies. The authors found 18 loci statistically significantly associated with AD and all of these had been reported by previous studies. Next, they combined this clinical AD data with the AD-by-proxy UK Biobank data. In all, 29 positions on the genome (known as loci) were found to be statistically significantly associated with AD, 9 of which were novel. Four of these nine were also found to be associated in the replication cohort (CLNK, ADAM10, APH1B and AC074212.3).
Using the full set of data from across all three phases, the authors then tested whether the genes found to be associated with AD were more likely than chance to be part of known sets of genes which represent particular biological functions (also known as enrichment). Four gene sets were associated with AD, all of which were in biologically plausible pathways relating to amyloid and lipids. There was also evidence of enrichment of genes in immune-related tissues.
Genetic risk associated with AD was found to correlate with genetic risk for a range of other medical conditions and non-medical traits. In addition, by using a technique called Mendelian Randomisation, the relationships between AD and cognitive ability, educational attainment and height were found to be causal.
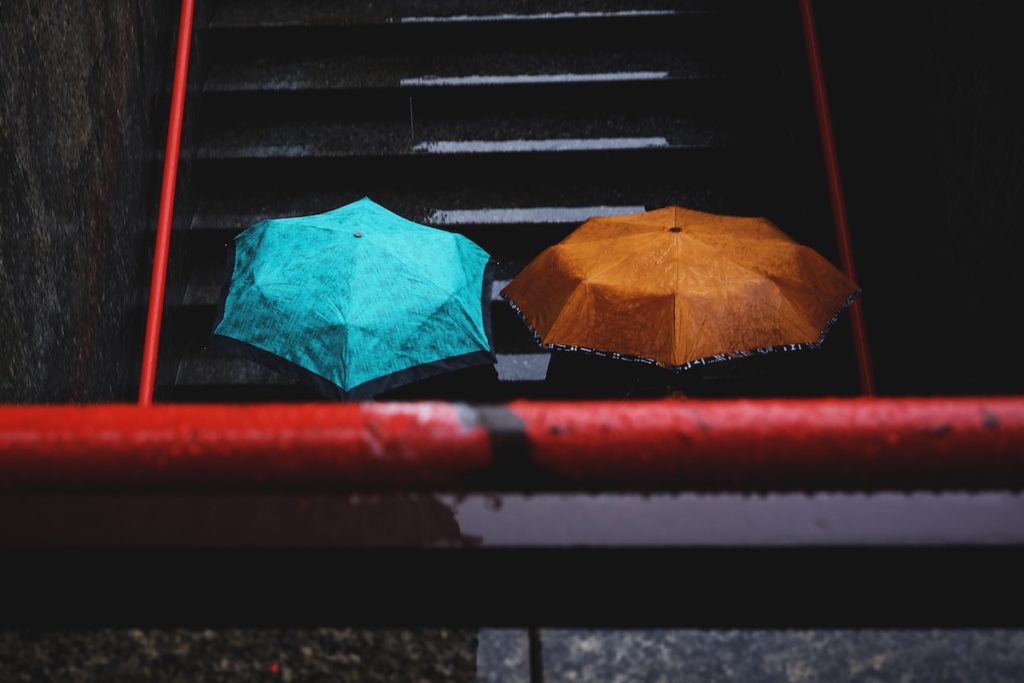
Cognitive ability, educational attainment and height were found to have a protective effect on Alzheimer’s disease.
Conclusions and implications for practice
This is a large, comprehensive study and as such only a portion of the results are summarised here. Overall, the authors conclude that the findings “strengthen the hypothesis that AD pathogenesis involves an interplay between inflammation and lipids” and also underscore the importance of the immune system in causing AD (rather than being a response to pathology) which could guide future research in the lab.
Directly translating any GWAS results into clinical practice is not easy and not always appropriate as we are often dealing with small effect sizes which offer little predictive value. Perhaps the most immediate translational aspects of this research are those relating to genetic correlation with other traits. These findings support clinical and epidemiological research showing protective effects of cognitive reserve and educational attainment on AD (Livingston et al. 2017). This is an interesting point which may guide further clinical studies targeting modifiable AD risk factors. For example, there is some evidence that ‘brain training’ may be beneficial to cognition in older adults (Corbett et al, 2015). Whether such training translates to dementia risk reduction remains to be seen but these genetic findings could provide support for closer examination of the impact of such interventions.
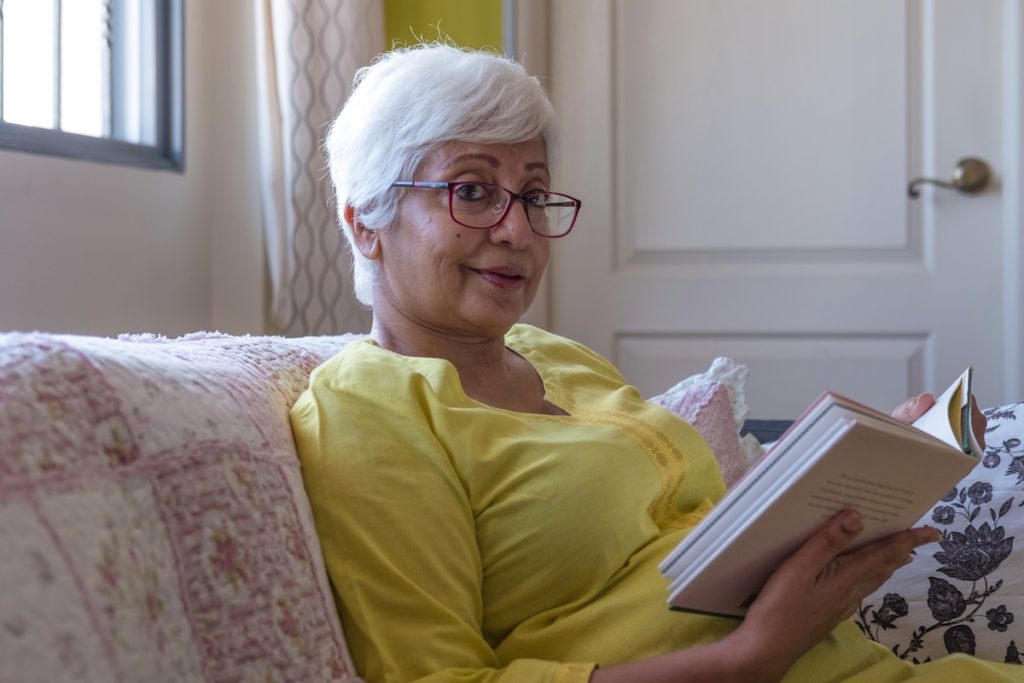
This research shows a causal link between cognitive ability and Alzheimer’s disease, which links in with epidemiological findings.
Strengths and limitations
This study implements a powerful discovery/replication design. The bioinformatic methods used to elucidate both functional pathways and causative relationships with other correlated traits mean we are provided with a comprehensive foundation to inform future research. And that is what is needed now because an inherent limitation of the GWAS approach is the limited insight gained into the molecular mechanisms underpinning disease.
A further limitation in the methods is the use of the proxy phenotype (family history of AD). It is a more noisy measure because it is indirect, but the authors demonstrate a strong genetic correlation between proxy and clinical AD cases status which justifies the approach. In addition, the wording of the question used to ascertain proxy status is worth mentioning. In the UK Biobank people are not asked specifically whether their relative had Alzheimer’s disease, they are asked about “Alzheimer’s/dementia”. While AD is the most common form of dementia by some margin, 20-40% of dementia cases are not AD and it is likely this question captures these individuals as well, though the effect of this more imprecise phenotype is likely mitigated by the very large sample size used. Moreover, the incorporation of the replication phase of the study, in which a cohort comprised of AD patients clinically diagnosed by specialists was used, adds confidence to the findings although it is possible that if other types of dementia are included among the ‘cases’ then some findings may not be specific to AD and some genuine AD associations may be masked.
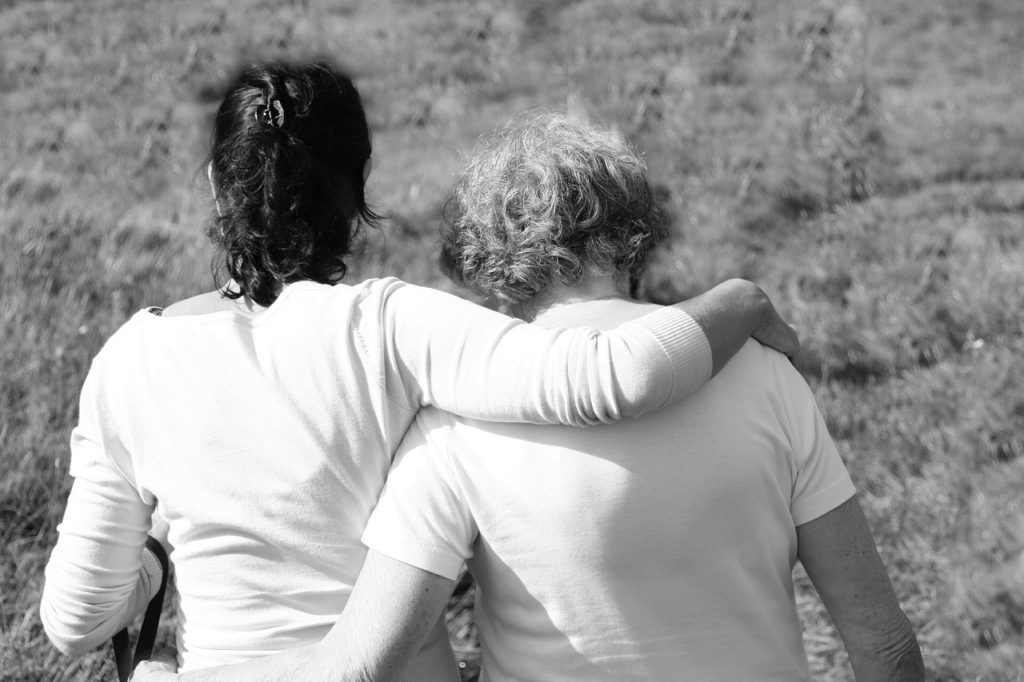
Much of the cohort used a proxy phenotype, whereby case/control status was assigned on the basis of family history. While this is a less precise measurement the authors used an additional cohort of clinically diagnosed AD to replicate the findings.
#LetsTalkMentalHealthII videos
#LetsTalkMentalHealthII is a series of videos created by the ESRC Mental Health Leadership Fellow (Prof Louise Arseneault) and her team, which aims to:
- Look ahead to the future for young people in mental health
- Highlight young people’s voice in mental health
- Promote the work of emerging disciplines in mental health
- Emphasise the perspectives of people with lived experience of mental health difficulties.
Join the #LetsTalkMentalHealthII conversation on Twitter or watch the videos on the Mental Health Leadership YouTube channel.
Conflicts of interest
Byron Creese is currently collaborating with Prof Ole Andreassen on other research.
Links
Primary paper
Jansen I, Savage J, Watanabe K. et al. (2019) Genome-wide meta-analysis identifies new loci and functional pathways influencing Alzheimer’s disease risk. Nature Genetics 51(3) 404-413. http://dx.doi.org/10.1038/s41588-018-0311-9 [PubMed abstract]
Other references
Harold D, Abraham R, Hollingworth P. et al (2009) Genome-wide association study identifies variants at CLU and PICALM associated with Alzheimer’s disease. Nature Genetics 41 1088-1093.
Lambert J-C, Ibrahim-Verbaas C, Harold D. et al (2013) Meta-analysis of 74,046 individuals identifies 11 new susceptibility loci for Alzheimer’s disease. Nature Genetics 45(12) 1452-1458.
Dementia statistics. Alzheimer’s Disease International website, last accessed 03 May 2019.
Alzheimer’s Society (2014) Dementia UK: Update.
Livingston G, Sommerlad A, Orgeta V. et al. (2017) Dementia prevention, intervention, and care. The Lancet 390(10113) 2673-2734.
Corbett A, Owen A, Hampshire A. et al. (2015) The Effect of an Online Cognitive Training Package in Healthy Older Adults: An Online Randomized Controlled Trial. JAMDA 16(11) 990-997.
Photo credits
- Photo by Elien Dumon on Unsplash
- Photo by Victor Vorontsov on Unsplash
- Photo by Luca Bravo on Unsplash
- Photo by BBH Singapore on Unsplash
Fine, fine, fine, fine, fine…
But let’s not focus on genes and meds all the time. I’m pretty sure there could be more cognitive science research as to how we could minimise, with cognitive exercises and social support, the cognitive blow of senility, broadly speaking.
‘ . . . dementia (Alzheimer’s Disease International, 2015), the most common cause of which is Alzheimer’s disease . . . ‘
This is a seriously INACCURATE statement. Alzheimer’s Disease does not CAUSE Dementia – it is one form of Dementia. If you are publishing, please strive for accuracy.